Abstract
Introduction: African swine fever virus (ASFV) causes African swine fever, a highly fatal hemorrhagic viral disease of domestic swine, severely impacting the development of swine industries in affected countries. The management of this disease is significantly hindered by the absence of protective vaccines against the virus. A cost-effective approach to developing potent vaccines is by employing immunoinformatic tools to identify highly conserved IFN-g , CD4+, CD8+, and B-cell epitopes. These can then be combined to create a multi-epitope broad-spectrum vaccine.
Methods: Therefore, in this study, immunoinformatic tools were utilized to identify CD8+ T-cell, CD4+ T-cell, B-cell, and IFN-g epitopes of the ASFV major coat protein p72, CD2 homologue (CD2v), and C-type lectin-like proteins, which are promising vaccine candidates capable of eliciting a protective immune response against the virus. The epitopes were computationally assembled to generate a multi-epitope subunit vaccine against ASFV. Molecular docking was employed to assess the interaction between the vaccine construct and immune receptors Toll-like receptor 9 (TLR-9) and Swine Leukocyte Antigen-1 (SLA-1).
Results: Molecular dynamic simulation revealed stable interactions between the vaccine construct and the immune receptors. In silico cloning and codon optimization were utilized to enhance the efficient expression of the vaccine in an E. coli expression system. The potential of the vaccine to provoke effective immune responses was evaluated using in silico immune simulation.
Conclusion: These computational approaches have shown that the vaccine is structurally stable and capable of inducing both humoral and cell-mediated immune responses against ASFV. However, this study needs to be validated further experimentally.
Introduction
African swine fever (ASF) is a highly contagious, fatal hemorrhagic disease that affects domestic pigs and wild boars (Sus scrofa), negatively impacting the development of the swine industry in affected countries. The disease was first described in Kenya in 19211 and is now enzootic in Sub-Saharan Africa, the Russian Federation, the Caucasus, Eastern Europe, and, most recently, China2, 3. Currently, there is no vaccine or effective therapeutics for proper management of this malady, and as such, culling infected pigs is the only method of disease control. The African swine fever pandemic has resulted in about a $2 billion loss to the swine industry worldwide. The causative agent, ASFV, belongs to the family Asfarviridae, the genus Asfarvirus, and it is the only known DNA-containing arbovirus4. The 200 nm-diameter African swine fever virus is a nucleocytoplasmic large double-stranded DNA virus. The virion's structure includes an enclosed nucleoid and core shell, icosahedral inner capsid, icosahedral membrane, outer capsid, and outer envelope5. A plethora of proteins contribute to the production of the outer capsid, including p72, p49, and p17, but p72, encoded by the B646L gene, is the main capsid protein of ASFV. It has been utilized to characterize the virus into genotypes and is a crucial target for the development of an ASF vaccine5. The outer envelope harbors the virus attachment protein p12, and the EP402R and EP153R gene products, CD2v and C-type lectin, respectively. CD2v is a homologue of CD2, a cell adhesion molecule expressed by T-lymphocytes (T-cells) and natural killer (NK) cells6. CD2v and C-type lectin proteins have been demonstrated to be responsible for the hemadsorption propensity of ASFV, and this has been employed in hemadsorption inhibition (HAI) serological typing of ASFVs into eight serogroups, although more serogroups might still be undetected. These serotype-specific proteins, CD2v and/or C-type lectin, have also been revealed to be crucial for defense against homologous ASF infection, indicating that ASF protective immunity may be serotype-specific. Since there is no effective vaccine against ASF currently, and taking into account the immunogenicity of p72, CD2v, and C-type lectin proteins, a pragmatic approach is the development of a multi-epitope vaccine against the virus. Multi-epitope vaccines are advantageous over classical vaccines for the following reasons: (I) They incorporate certain IFN-epitopes and other adjuvant-capable innate immune response components, which can improve immunogenicity and long-lasting immune responses; (II) they consist of cytotoxic T-lymphocytes (CTL), T-helper (Th), and B-lymphocyte (B-cell) epitopes, which could resolve the issue of various ASFV serotypes/genotypes in vaccine development; (III) they reduce unwanted components that can provoke hypersensitivity and immunopathologic responses, and very importantly; (IV) they contain several MHC-restricted epitopes that can be detected by T-cell receptors (TCRs) from various clones from different T-cell subsets. The presentations of viral peptides in the context of MHC class I and class II are essential for anti-viral T-cell responses. Unlike B-cell epitopes responsible for eliciting neutralizing antibodies, T-cell epitopes can be located anywhere in a viral protein since cells can process and present both intracellular and extracellular viral peptides. The majority of MHC class I-restricted T-cell epitopes originate from intracellular antigens that are cleaved by the proteasome and transported into the endoplasmic reticulum by the transporter associated with antigen processing proteins (TAP), where they can bind to MHC class I molecules, which are then transported to the cell surface for recognition by CD8+ T-cells. However, extracellular proteins picked up by professional antigen-presenting cells and cleaved in lysosomes are the main source of MHC class II-restricted T-cell epitopes. These proteins can attach to MHC class II molecules, be transported to the cell surface, and then be recognized by CD4+ T cells7. A vaccine-induced CD8+ T-cell-mediated immune response might be long-lived and cross-serotype and thus deserves further attention. The swine MHC, designated swine leukocyte antigen (SLA), consisting of SLA class I loci (SLA-1, SLA-2, SLA-3) and SLA class II loci (DRB1, DQB1, DQA)8, 9, has been shown to be involved in swine immune response to various viral infections such as Foot-and-mouth disease virus and influenza-A viruses. This is possible because of the presence of six pockets (A to F) in the peptide-binding groove (PBG) of SLA-1 that the viral peptides could bind10. Since the mainstay of developing an efficacious multi-epitope vaccine is the selection of appropriate candidate antigens and their immunodominant epitopes, these sites can be easily selected using immunoinformatics. Therefore, the immunoinformatics approach is a good alternative for streamlining a cheap and straightforward experimental approach, since the experimental selection of B- and T-cell epitopes is time-consuming and expensive. Immunoinformatics employs computational methods such as machine learning, artificial neural networks, and deep learning to predict B- and T-cell epitopes. Herein, B- and T-cells of ASFV CD2v, C-type lectin, and p72 epitopes were predicted and validated using immunoinformatic methods. These epitopes were employed to construct and validate the ASFV multi-epitope vaccine. These findings may provide a basis for the development of other multi-epitope vaccines.
Methods
Protein sequences
The amino acid sequences of CD2v, C-type lectin, and p72 of all serotypes/genotypes of ASFV were retrieved from GenBank. The retrieved sequences for each protein were aligned within serotypes using Geneious software. The consensus sequences of each protein were subjected to Blast-p analysis (http://blast.ncbi.nlm.nih.gov/Blast.cgi). The best matches for each protein sequence, CD2v (AJB28366), C-type-like protein (AAC28412), and p72 (QID21249), were selected for epitope screening. The antigenicity (at a threshold of 0.4) of selected proteins was evaluated via VaxiJen v2.0 (http://www.ddg-pharmfac.net/vaxijen/VaxiJen/VaxiJen.html)11.
T-cell and B-cell epitope prediction
An array of tools exists for predicting epitopes that could be presented by MHC on host cells to T-cells. The Immune Epitope Database and Analysis Resource (IEDB), a web-based epitope analysis resource that includes tools for T cell and B cell epitope prediction, was utilized. This resource employs artificial neural network (ANN), stabilized matrix method (SMM), and combinatorial peptide libraries (CombLib) to predict the natural process and presentation of peptides by MHC I. IEDB MHC-I NetMHCpan EL 4.1 has primarily been trained on existing MHC–peptide binding data from chimpanzee, cow, gorilla, human, macaque, mouse, pig, dog, and horse to predict the peptide-binding specificity of MHC molecules. It was used to screen the extracellular domains of CD2v (amino acid positions 17-204), C-type lectin (amino acid positions 52-161), and p72 as epitopes for SLAs that would be presented to CD8+ T-cells (CTLs). For SLA-II (HLA-II) alleles, which were employed to select peptides for presentation to CD4+ T-cells since SLA-II is not present in IEDB, it has been demonstrated that HLA-II can serve as a proxy for other mammals12. Furthermore, SLA-II genes have shown much stronger sequence similarity with their HLA-II counterparts than with each other13. The IEDB-recommended method2.22 was used to screen for the aforementioned proteins for potential peptides as epitopes for MHC-II (HLA-II) that would be presented to CD4+ T-cells (HTLs). The ABCpred server (http://crdd.osdd.net/raghava/abcpred/) was utilized for B-cell epitope prediction, which is based on ANN for predicting linear epitopes.
Conformational/discontinuous B-cell epitopes were predicted from the proteins using IEDB Discotope 2.0 (http://tools.iedb.org/discotope/) with default parameters14. The discontinuous epitopes were selected against the resolved structure of ASFV p72 (PDB:6KU9). Epitopes were chosen based on promiscuity, antigenicity, and non-toxicity criteria. The antigenicity of selected epitopes was verified by VaxiJen v2.0 (at a threshold of 0.4)11. ToxinPred (http://crdd.osdd.net/raghava/toxinpred/) was used to predict the nontoxic/toxic properties of epitopes15.
IFN-γ epitope prediction
IFN-γ plays a critical role in antiviral, anti-tumor, and immune regulatory activities. It triggers both innate and adaptive immune responses by activating macrophages and NK cells16. Thus, identifying IFN-γ inducing epitopes is crucial for designing a potential multi-epitope vaccine. The IFNepitope server (https://crdd.osdd.net/raghava/ifnepitope/) was used for predicting IFN-γ epitopes in the target proteins.
Multi-epitope subunit vaccine construction, structural modeling, refinement, and validation
The vaccine was constructed by linking Suis scrofa β-defensin, the selected CTL, HTL, B-cells, and IFN-γ inducing epitopes, along with the TAT protein together via linkers such as EAAAK, GPGPG, KK, and AAY. β-defensins, endogenous alarmins/antimicrobial peptides, are responsible for promoting both local innate and adaptive systemic immune responses against pathogens. They play roles in immune responses by inducing innate immune cells and recruiting naïve T-cells through the chemokine receptor-6 (CCR-6)17. This antimicrobial peptide was appended at the N-terminal of the vaccine construct to enhance the immune response provoked by the vaccine. At the C-terminal of the vaccine construct, a 12-mer cell-penetrating peptide with residues GRKKRRQRRRPQ, derived from the Human immunodeficiency virus-1 (HIV-1) transactivator of transcription (TAT) protein18, was grafted. This peptide, with cell-penetrating properties, will assist the vaccine construct in intracellular delivery and sub-cellular localization. The amino acid residues of β-defensin at the N-terminal of the vaccine construct were linked with CTL epitopes via EAAAK linkers, and the CTL epitopes were linked through AAY linkers. CTL epitopes were joined with HTL epitopes by GPGPG, while B cells, IFN-γ, and the TAT protein were linked via KK linkers. These linkers are essential for protein folding, flexibility, and separation of functional domains of the vaccine construct. A Protein BLAST search was employed using default parameters to ensure that the vaccine construct is not homologous to the pig proteome. Proteins with less than 37% identity with 100% coverage are regarded as non-homologous. The physicochemical parameters of the vaccine construct were determined using the ProtParam tool (http://web.expasy.org/protparam/)19. ProtParam predicts various physicochemical parameters such as the number of amino acids, molecular weight, theoretical isoelectric point (pI), amino acid composition, atomic composition, formula, extinction coefficients, estimated half-life, instability index, aliphatic index, and the GRand AVerage of hydropathY (GRAVY). The VaxiJen v2.0 server (http://www.ddgpharmfac.net/vaxijen/VaxiJen/VaxiJen.html) was used to predict the antigenicity of the vaccine construct through an alignment-free approach based on autoregressive moving average_cross_mean absolute deviation (ARMA-MAD) transformation of protein sequences into uniform vectors of principal amino acid properties11. A key feature of the vaccine is that it should be non-allergenic, as allergenic proteins can induce hypersensitivity in the host. Therefore, the vaccine must be non-allergenic. The AlgPred server (http://www.imtech.res.in/raghava/algpred/) was used to determine the allergenicity of the vaccine construct. A hybrid approach (SVMc+IgE epitope+ARPs BLAST+MAST) in AlgPred was utilized to predict the allergenicity of the vaccine construct based on its high accuracy and sensitivity20. The tertiary or three-dimensional (3D) structure of the vaccine construct from its linear structure was generated using a transform-restrained Rosetta (trRosetta) algorithm, which predicts protein 3D structures based on predicted inter-residue orientations through a deep learning-based method21. The predicted 3D structure was refined using ModRefiner, an algorithm used for atomic-level, high-resolution protein structure refinement, which can start from either a C-alpha trace, a main-chain model, or a full-atomic model22. Thereafter, the 3D structure was validated using the ERRAT score at the ERRAT server (https://servicesn.mbi.ucla.edu/ERRAT/), which evaluates the calculation of non-bonded interactions in the vaccine construct structure. The overall quality of the generated model of the vaccine construct was also validated by PDBSum Generate (https://www.ebi.ac.uk/thornton-srv/databases/pdbsum/Generate.html) for Ramachandran plot analysis and G-factor determination. The Ramachandran plot reveals favored regions for backbone dihedral angles Phi (ϕ) and Psi (ψ) against amino acid residues in protein structure23. This was followed by ProSA-web analysis to validate the structure based on the Z-score prediction.
Molecular docking of the vaccine construct with swine immune receptors
Germline-encoded pattern recognition receptors (PRRs) such as toll-like receptors (TLRs) and inflammasomes play a pivotal role in inducing an anti-viral state in the host through the innate immune response via distinct signaling pathways, resulting in the production of pro-inflammatory cytokines, chemokines, and interferons24. Moreover, TLRs activate antigen-presenting cells (APCs) to work in concert with adaptive immunity for pathogen elimination and induction of long-term immunity25. These receptors recognize conserved viral molecular patterns (PAMPs) and subsequently provoke an immune response in the host if an antigen or vaccine interacts appropriately with the target immune cells. Toll-like receptors are type 1 transmembrane horseshoe-shaped proteins that recognize PAMPs and signal via MyD88-dependent or TRIF-dependent pathways. TLRs 1, 2, 4, 5, and 6 are located on the cell surface, whereas TLRs 3, 7, 8, and 9 are situated on endosomal membranes and detect viral nucleic acids26. TLR9, in particular, recognizes double-stranded DNA containing unmethylated CpG motifs in endosomes and has been found to induce innate immunity in herpesvirus infections. Therefore, molecular docking analysis was carried out to demonstrate the binding affinity between the vaccine construct and immune receptors TLR-9 (TLR-9, PDB:3wpb) and SLA-1 (SLA-1*0401, PDB:3qq4). The docking of the vaccine construct with TLR-9 and SLA-I receptors was performed using High Ambiguity Driven protein-protein DOCKing (HADDOCK) 2.4 (https://www.bonvinlab.org/software/haddock2.4/)27. The best cluster was selected from the docked clusters based on the lowest HADDOCK score. Thereafter, the HADDOCK Refinement Interface was employed to refine the chosen cluster. The best structure after refinement from each docked complex was chosen, and their binding affinity was determined using the PROtein binDIng enerGY prediction (PRODIGY) web server28. PRODIGY employs a simple but powerful predictive model based on intermolecular contacts and properties derived from non-interface surfaces to predict the binding affinity of protein-protein complexes through their 3D structure29. Finally, the interacting residues between the vaccine construct and the immune receptors were mapped using PDBsum (https://www.ebi.ac.uk/thornton-srv/databases/pdbsum/Generate.html)30.
Molecular dynamics simulation
Molecular dynamics simulation was performed using the inbuilt commands of GROMACS (GROningen MAchine for Chemical Simulations) to evaluate the structural properties and interactions in vaccine construct-TLR-9 and vaccine construct-SLA-1 complexes. The Assisted Model Building and Energy Refinement 03 (AMBER03) force field was used to create the topology file essential for energy minimization and equilibration. The spc/e water model was employed as the solvent to simulate the complex with periodic boundary conditions. The net charge of the complex was determined, and charged ions were added to neutralize the system. Energy minimization was carried out before simulation to ensure the adequate geometry of the system and the absence of steric clashes using the steepest descent algorithm approach. The trajectories generated from the simulation (10 ns) were analyzed for the stability of the energy-minimized complex in terms of root mean square deviation (RMSD) and root mean square fluctuation (RMSF) of backbone atoms and side chains, respectively.
Codon optimization and in silico cloning
To generate an appropriate plasmid construct harboring the vaccine construct sequence, codon optimization was embarked upon. Codon optimization is a method to achieve translation efficiency of foreign genes in the host if the use of codons differs between the two organisms. The Java Codon Adaptation Tool (JCat) (https://www.jcat.de/) was used for codon optimization to generate a DNA sequence of the vaccine construct that can be efficiently expressed in the E. coli K-12 strain31. The Codon Adaptation Index (CAI), a technique for analyzing codon usage bias32, and the guanine and cytosine (GC) contents were evaluated. To ensure translation efficiency, the RNAfold web server was employed to predict the secondary structure of the optimized codon sequences. Sticky ends of the restriction sites for BamHI and EcoRI restriction enzymes were grafted to allow restriction and cloning at the start (N-terminal) and end (C-terminal) of the modified vaccine sequence, respectively. The modified nucleotide sequence of the vaccine was cloned into the E. coli pET-28a (+) vector using the SnapGene tool (https://www.snapgene.com/) to ensure its in vitro expression.
Immune Response Simulations
To predict and characterize the immune response profile of the vaccine construct in the host, computational immune simulations were employed by the C-IMMSIM server (http://kraken.iac.rm.cnr.it/C-IMMSIM/)33. The C-ImmSim uses the Celada-Seiden model to describe both humoral and cell-mediated immune profiles of a mammalian immune system against a designed vaccine. C-IMMSIM is an agent-based model that employs position-specific scoring matrices (PSSM) derived from machine learning techniques for predicting immune interactions. The summary of the pipeline for vaccine development is described (Figure 1).
Protein | SLA I | |||
CD2v | Peptides (positions) | Alleles | Antigenicity | Toxicity |
TNKSFLNYYW (151-160) | SLA-2*0402, SLA-1*0501, SLA-2*0401 | 0.5346 | Non-toxic | |
C-type lectin | KYTGLIDKNY (6-15) | SLA-2*0102, SLA-1*0501 | 1.1132 | Non-toxic |
FSNNIDEKNY (81-90) | SLA-2*1002 | 0.4413 | Non-toxic | |
KKYNYESGYW (116-125) | SLA-1*0501, SLA-3*0701, SLA-3*0301, SLA-3*0303, SLA-3*0401 | 1.0042 | Non-toxic | |
KKVNYTGLLF (145-154) | SLA-1*0401, SLA-3*0601, SLA-3*0301, SLA-3*0303, SLA-3*0304, SLA-1*0801, SLA-3*0701 | 1.2916 | Non-toxic | |
p72 | YGKPDPEPTL (40-49) | SLA-1*1101, SLA-3*0101, SLA-2*0701, SLA-2*0501, SLA-3*0501, SLA-3*0502, SLA-3*0503, SLA-6*0101, SLA-6*0102, SLA-6*0103, SLA-6*0104, SLA-6*0105 | 1.4480 | Non-toxic |
KPYVPVGFEY (65-74) | SLA-1*0701, SLA-1*0702, SLA-2*0102, SLA-2*0101, SLA-1*0501, SLA-2*0302, SLA-2*0401, SLA-2*0402, SLA-3*0601, SLA-1*0201, SLA-1*0202, SLA-1*0801, SLA-1*0601, SLA-1*1301, SLA-3*0701, SLA-2*1001, SLA-2*1002, SLA-2*0601, SLA-2*0301, SLA-1*0702, SLA-1*0401, SLA-1*0101, SLA-3*0304, SLA-3*0301, SLA-3*0303, SLA-3*0302 | 1.3466 | Non-toxic | |
SVSIPFGERF (347-356) | SLA-1*1201, SLA-1*0201, SLA-1*0202, SLA-2*0302, SLA-1*0601, SLA-2*0501, SLA-2*0101, SLA-1*0401, SLA-1*0501, SLA-2*1001, SLA-1*1301, SLA-2*0401, SLA-1*0801, SLA-2*0502, SLA-2*1002, SLA-2*1201, SLA-2*0402, SLA-2*0102, SLA-3*0302 | 0.8502 | Non-toxic | |
SRRNIRFKPW (387-396) | SLA-3*0701, SLA-3*0301, SLA-3*0303, SLA-3*0304, SLA-3*0401, SLA-3*0601, SLA-2*0402 | 1.9015 | Non-toxic | |
SISDISPVTY (521-530) | SLA-2*1002, SLA-1*0401, SLA-2*0302, SLA-1*1301, SLA-1*0801, SLA-1*0601, SLA-2*1001, SLA-2*0401, SLA-1*0201, SLA-1*0202, SLA-1*0701, SLA-1*0702, SLA-1*1201, SLA-2*0101, SLA-2*0102, SLA-1*0501, SLA-2*0402, SLA-1*0101, SLA-3*0302 | 1.5700 | Non-toxic |
MHC II | ||||
Protein | Peptides (position) | Alleles | Antigenicity | Toxicity |
CD2v | LVYSRNRINYTINLL (96-110) | HLA-DRB3*02:02, HLA-DRB3*01:01, HLA-DRB1*15:01, HLA-DRB1*07:01, HLA-DRB1*03:01, HLA-DRB5*01:01, HLA-DRB4*01:01 | 0.8727 | Non-toxic |
HLA-DPA1*01:03/DPB1*08:01, HLA-DPA1*01:03/DPB1*05:01, HLA-DPA1*01:03/DPB1*01:01, HLA-DPA1*01:03/DPB1*02:02, HLA-DPA1*01:03/DPB1*06:01, HLA-DPA1*01:03/DPB1*03:01, HLA-DPA1*01:03/DPB1*04:02. | ||||
HLA-DQA1*01:01/DQB1*02:05, HLA-DQA1*01:01/DQB1*02:01, HLA-DQA1*01:01/DQB1*02:02, HLA-DQA1*01:01/DQB1*02:04, HLA-DQA1*01:01/DQB1*02:06, HLA-DQA1*01:01/DQB1*02:03, HLA-DQA1*01:01/DQB1*03:02. | ||||
C-type like lectin | TKKYNYESGYWVNYS (115-129) | HLA-DRB3*01:01, HLA-DRB1*15:01, HLA-DRB3*02:02, HLA-DRB1*07:01, HLA-DRB5*01:01, HLA-DRB1*03:01, HLA-DRB4*01:01 | 1.0513 | Non-toxic |
HLA-DPA1*01:03/DPB1*04:01, HLA-DPA1*02:01/DPB1*105:01, HLA-DPA1*03:02/DPB1*04:01, HLA-DPA1*04:01/DPB1*126:01, HLA-DPA1*01/DPB1*04:01, HLA-DPA1*01:03/DPB1*16:01, HLA-DPA1*02:03/DPB1*17:01 | ||||
HLA-DQA1*05:01/DQB1*05:06, HLA-DQA1*03:03/DQB1*06:28, HLA-DQA1*01:02/DQB1*03:14, HLA-DQA1*05:07/DQB1*03:22, HLA-DQA1*04:02/DQB1*06:37, HLA-DQA1*06:02/DQB1*06:29 | ||||
p72 | HHAEISFQDRDTALP (502-516) | HLA-DRB4*01:01, HLA-DRB3*01:01, HLA-DRB1*03:01, HLA-DRB1*15:01, HLA-DRB3*02:02, HLA-DRB1*07:01, HLA-DRB5*01:01 | 2.0206 | Non-toxic |
HLA-DPA1*01:06/DPB1*76:01, HLA-DPA1*02:04/DPB1*41:01, HLA-DPA1*03:01/DPB1*109:01, HLA-DPA1*01:03/DPB1*03:01, HLA-DPA1*01/DPB1*04:01, HLA-DPA1*04:01/DPB1*134:01, HLA-DPA1*04:01/DPB1*131:01 | ||||
HLA-DQA1*01:01/DQB1*02:01, HLA-DQA1*01:04/DQB1*04:03, HLA-DQA1*01:02/DQB1*05:12, HLA-DQA1*01:01/DQB1*03:09, HLA-DQA1*01:05/DQB1*03:09, HLA-DQA1*01:03/DQB1*06:42, HLA-DQA1*01:06/DQB1*03:17 |
Protein | Peptides (position) | Score | Antigenicity | Toxicity |
CD2v | SLITCEKTNGTNIR (125-138) | 0.68 | 0.6030 | Non-toxic |
C-type lectin | NDTNLLNLTKKYNY (107-120) | 0.87 | 1.1367 | Non-toxic |
p72 | CSHTNPKFLSQHFP (268-281) | 0.68 | 0.6627 | Non-toxic |
DITPITDATYLDIR (294-307) | 0.58 | 1.7313 | Non-toxic |
Protein | Peptides (position) | Antigenicity | Toxicity |
p72 | FPGLFVRQSRFIAGR (371-385) | 0.4942 | Non-toxic |
SRRNIRFLPWFIPGV (387-401) | 1.5164 | Non-toxic |
Protein | Peptides (position) | Score | Antigenicity | Toxicity |
CD2v | TVTLNSNINSETEGI (25-40) | 12 | 0.2457 | Non-Toxic |
C-type lectin | ESGYWVNYSLANNQS (120-135) | 1 | 0.7730 | Non-Toxic |
p72 | SNIKNVNKSYGKPDP (30-45) | 6 | 1.0862 | Non-Toxic |
Vaccine construct-Receptor complex | Gibbs free energy (kcal mol-1) | Kd (M) |
Vaccine construct-TLR‐9 | -17.0 | 3.5E-13 |
Vaccine construct-SLA-1 | -8.9 | 3.1E-07 |
Results
Analysis of the selected three structural proteins
The best consensus sequences of CD2v (AJB28366), C-type like protein (AAC28412), and p72 (QID21249) after multiple sequence alignments and Blast-p analysis were used for screening epitopes using VaxiJen v2.0. The antigenicity scores from VaxiJen v2.0 showed all three proteins are antigenic. p72 is the most antigenic with a score of 0.5063 followed by the C-type lectin protein with 0.4583 and CD2v with 0.4171.
T-cell epitopes predictions
The selection of highly immunogenic CTL and HTL epitopes is very important for the vaccine to produce long-lasting immunity. In particular, a CTL response is more important for effective viral clearance. HTL epitopes play a crucial role in generating both humoral and cellular immune responses. The extracellular domains of CD2v, C-type lectin, and p72 were submitted to the IEDB server to predict (10mer) CTLs and (15mer) HTLs epitopes, applying the IEDB recommended 2020.04 (NetMHCpan EL 4.0) and IEDB recommended 2.22 (Consensus) prediction methods, respectively. Predicted epitopes with strong binding affinities (based on low percentile rank) were further screened using allele criterion promiscuity, antigenicity, and non-toxicity to select the best epitopes. Using these criteria, 10 SLA-I (CD2v-1, C-type lectin-4, and p72-5) and 3 MHC-II (CD2v-1, C-type lectin-1, and p72-1) epitopes were selected (Table 1, Table 2).
B-cell epitopes predictions
Linear B-cell epitopes were selected using the ABCpred server whereas discontinuous B-cell epitopes were predicted from the proteins using IEDB Discotope 2.0. The predicted linear B-cell epitopes were ranked according to their scores obtained by a trained recurrent neural network. A higher score of the peptide indicates a higher probability of being an epitope. From the 14mers generated from input sequences, 6 B-cells (CD2v-1, C-type lectin-4, and p72-2 as linear epitopes; p72-2 as discontinuous epitopes) were shortlisted based on their scores and VaxiJen antigenicity (Table 3, Table 4).
IFN-γ epitopes prediction
The IFNepitope server was employed to predict IFN-γ epitopes. Predicted epitopes with strong binding affinities (based on low percentile rank) were further screened using allele criterion promiscuity, antigenicity, and non-toxicity to select the best epitopes. Using these criteria, 3 IFN-γ epitopes (CD2v-1, C-type lectin-1, and p72-1) were selected (Table 5).
Multi-epitope subunit vaccine construction, structural modeling, and validation
The vaccine construct was developed by joining epitopes with the highest prediction scores. A total of 22 epitopes comprising 10 CTL epitopes, 3 HTL, 6 B-cell epitopes, and 3 IFN-γ epitopes were selected based on their prediction score and were joined together using their corresponding linkers: HTL epitopes, B-cell epitopes, IFN-γ, and CTL epitopes were joined together using GPGPG, KK, and AAY linkers, respectively. To boost the vaccine construct's immunogenicity, a 45 amino acid-long sequence of Sus scrofa β-defensin was added as an adjuvant at the N-terminal of the construct with the aid of EAAAK linker. EAAAK linkers aid in limiting the interaction of the adjuvant with other protein regions, efficiently separating and bolstering stability. At the C-terminal end, a TAT protein, which is cell-penetrating, was appended to the construct to help in intracellular delivery (Figure 2 A). The final vaccine construct was 437 amino acid residues long and was visualized using PyMol (Figure 2 B).
Vaccine construct’s physicochemical parameters
ExPASy ProtParam tool revealed various physicochemical properties of the vaccine construct. The construct, composed of 437 amino acid residues with a molecular weight of 48991.45 g/mol, theoretical pI of 9.84, reveals that the vaccine construct is basic. The instability index (II) score of 34.91 classifies the vaccine construct as being stable in nature since values above 40 are regarded as unstable. The GRAVY index of -0.708 verifies the hydrophilic nature of the vaccine construct, revealing that it can form interactions with surrounding water molecules. The aliphatic index of 59.70 shows that the construct is thermostable. The estimated half-life was 1 h (mammalian reticulocytes, in vitro), 30 min (yeast, in vivo) and more than 10 hours (E. coli, in vivo). The antigenicity of the vaccine construct was predicted using the VaxiJen server and was found to be a good antigen with a score of 0.6782 with the default set threshold value of 0.4 for viruses. Furthermore, the AlgPred server was employed to determine the allergenicity of the vaccine construct using a hybrid approach (SVMc+IgE epitope+ARPs BLAST+MAST). The vaccine construct was observed to be non-allergenic. The 3D structure of the vaccine construct was modeled from its linear structure using trRosetta; then, the modeled structure was refined using ModRefiner. Thereafter, the 3D structure was validated using an ERRAT score at the ERRAT server (https://servicesn.mbi.ucla.edu/ERRAT/), which evaluates the calculation of non-bonded interactions in the vaccine construct structure. Ramachandran plot analysis was also generated through the ERRAT server. This was proceeded by ProSA-web analysis to further validate the structure based on the predicted Z-score.
Molecular docking of the vaccine construct (ligand) with immune receptors (TLR‐9) and SLA-1
To optimize the interaction affinity between the vaccine construct and TLR-9 (PDB:3WPB)/SLA-1 (PDB: 3QQ4), molecular docking was carried out using the Haddock 2.4 server. For the vaccine construct-TLR-9 interaction, the server clustered 138 structures into 12 cluster(s), which represents 69% of the water-refined models generated. The top cluster is the most reliable according to HADDOCK. Its Z-score of -1.6 indicates how many standard deviations from the mean score. The HADDOCK score was -30.2 ± 8.2. The best cluster was selected from the docked clusters based on the lowest HADDOCK score. Thereafter, HADDOCK Refinement Interface was employed to refine the chosen cluster, wherein HADDOCK clustered 10 structures into 1 cluster(s), which represents 100% of the water-refined models HADDOCK generated. The top cluster had a Z-score of 0.0 and a HADDOCK score of -171.8 ± 4.1. Docking of the vaccine construct-SLA-I receptors was also carried out using HADDOCK 2.4. HADDOCK clustered 154 structures into 6 cluster(s), which represents 77% of the water-refined models HADDOCK generated. The top cluster had a Z-score of -1.7 and a HADDOCK score of -84.0 ± 5.9. When HADDOCK Refinement was carried out, HADDOCK clustered 10 structures into 1 cluster(s), which represents 100% of the water-refined models HADDOCK generated. The top cluster had a Z-score of 0.0 and a HADDOCK score of -106.5 ± 3.0. The best structure after refinement from each docked complex was chosen, and their binding affinity was determined using the PRODIGY web server (Table 6). The low binding energy signifies the formation of a strong complex. Docking interaction between the vaccine construct and TLR-9 revealed 14 H-bonds and 5 salt bridges that are involved in the interaction. Similarly, docking analysis between the vaccine construct and SLA-1 uncovered 14 hydrogen bonds and 4 salt bridges formed during the interaction. Also, in the docked vaccine construct-SLA-1 complex, the vaccine construct interactions are mainly with the PBG pockets A, E, and F. In pocket A, the vaccine construct K427 interacted with the SLA-1 E63 through an H-bond and salt bridge; L163 of the vaccine construct interacted with L163 through non-bonded interactions. In the E pocket, E416 of the vaccine construct interacted with R156 through an H-bond and salt bridge; also, R413 of the vaccine construct interacted with E152 and R114 through a salt bridge and non-bonded interactions, respectively. In the F pocket, the vaccine construct's P405 interacted with T80 through an H-bond and non-bonded interaction, while P406 interacted with K146 through H-bonding. Also in the F pocket, T398 of the vaccine construct bond to T73 through an H-bond, R413 of the vaccine construct bonded to T74 through an H-bond and non-bonding interactions, with T147 through non-bonded interactions and R114 through non-bonded interaction (Figure 3 B).
Molecular dynamics simulation
To estimate the stability of the vaccine construct-immune receptor complexes, molecular dynamics simulation of the refined docked complexes (vaccine construct-TLR-9 and vaccine construct-SLA-1) was carried out using GROMACS. The compactness of the two simulation systems around their protein axes was revealed by the radius of gyration plots. The vaccine construct-TLR-9 and vaccine construct-SLA-1 complexes had mean gyration values of 4.0 nm and 4.5 nm, respectively (Figure 4 A-B). This result indicates a relatively stable folded structure of the two complexes. For the density of the docked complexes, the vaccine construct-TLR-9 simulation system had 1007.78 kg/m3 with a total drift of 0.086 kg/m3, while that of the vaccine construct-SLA-1 was 1000.69 kg/m3 with a total drift of 0.073 kg/m3 (Figure 4 C-D). The temperature and pressure of the simulation system during the production run were around 300 K and 1.3 bar, respectively for the two complexes, showing a stable system and successful molecular dynamics simulation run (Figure 4 E–H). The RMSD of the vaccine construct-TLR-9 complex had some fluctuations during the simulation (Figure 4 I). The vaccine construct-SLA-1 had fluctuations 0-4 ns, thereafter, it became stable and the RMSD value remained around 1.1 nm, indicating that the conformation of this complex was stable (Figure 4 J). RMSF plots for the two complexes had high peaks indicating degrees of flexibility in the vaccine construct (Figure 4 K-L).
Codon optimization of the vaccine construct and in silico cloning
Codon adaptation carried out using JCat was used to optimize the codon usage of the vaccine construct in E. coli (strain K12) and to obtain the gene sequence of the vaccine construct. The Codon Adaptation Index was 0.92, GC content was 53.01%, and the optimized codon sequences were 1311 nucleotides long. To achieve good protein expression, the GC content is expected to be in the range of 30%-70%; therefore, the GC content of 53.01% is indicative of a good expression of the vaccine construct in E. coli. To ensure translation efficiency, the RNAfold web server was employed to predict the secondary structure of the optimized codon sequences. The predicted secondary structure of the optimized sequence was found to be stable, and it lacked any hairpin loop or pseudoknot at its starting site (Figure 5 A). Certain codon features like restriction enzyme cleavage sites (BamHI and EcoRI) and prokaryotic ribosome binding sites in the E. coli plasmid pET-28(+) were also taken into consideration using the SnapGene software (https://www.snapgene.com/) to ensure successful expression of the gene sequence. The whole clone was 6665 bp in size (Figure 5 B). For immune simulation, an antibody response was observed with high levels of IgM and IgG antibodies, which is common in virus vaccinations (Figure 5 C). Also, high levels of cytokines, T-helper cells, and cytotoxic T-cells were observed (Figure 5 D-F). These observations indicate evidence of a potent immune response that would be induced by the vaccine construct.
Discussion
African swine fever is a devastating disease affecting domestic and wild pigs, with serious socioeconomic consequences in affected countries. It has been over 100 years since it was first reported1, yet the disease continues to ravage swine populations due to the lack of effective vaccines and control measures. The development of an effective vaccine against ASF is hampered by the various genotypes and serotypes of the causative virus. Therefore, the development of an effective and affordable ASF vaccine using conventional approaches is not a short-term project. Several efforts have been made to develop ASFV vaccines using conventional methods. For instance, a genetically modified ASFV live attenuated vaccine was developed by deleting the I177L gene from the genome of a highly virulent ASFV strain34. Similarly, Ramirez-Medina et al.35 developed a vaccine candidate by deleting the E184L gene from the highly virulent ASFV Georgia 2010 (ASFV-G) isolate, resulting in reduced ASFV virulence during pig infections. However, the development of effective and cost-efficient ASF vaccines can be streamlined using immunoinformatics approaches. This approach involves screening the viral proteome to select immunogenic epitopes that induce highly targeted immune responses. These immunogenic peptides can be joined to produce a multiepitope vaccine. The multiepitope vaccine designed in this study offers advantages over live attenuated vaccines and other recombinant vaccines because it combines the main epitopes of the virus without peptides capable of inducing allergic reactions or other immunopathologies. The peptides in the vaccine construct are capable of inducing innate, humoral, and cell-mediated immune responses (Figure 5). Although there are various reports on the use of immunoinformatics to develop vaccines against diseases, especially COVID-1936, 37, there are no reports yet on ASF. Consequently, this study was embarked upon to design a multiepitope vaccine against ASFV using immunoinformatics tools. Vaccine candidate peptides were selected from HTL, B cell, IFN-γ, and CTL epitopes from conserved amino acid sequences of ASFV immunogenic proteins CD2v, C-type lectin, and p72 based on their antigenicity, toxicity, and allergenicity. The observation of two motifs, CSHTNPKFLSQHF and DITPITDATY, on the p72 immunogenic peptides 268-268-CSHTNPKFLSQHFP-281 and 294-DITPITDATYLDIR-307 selected from this study, as well as the experimental p72 monoclonal antibody-mapped epitopes 265-QRTCSHTNPKFLSQHF-280 and 290-AGKQDITPITDATY-303, validated our immunogenic peptide selection procedure38, 39. The vaccine construct was developed by joining selected HTL epitopes, B-cell epitopes, IFN-γ, and CTL epitopes together using GPGPG, KK, and AAY linkers, respectively. Joining the epitopes with linkers mimics the structure of naturally-occurring multi-domain proteins, decreasing the chance of junctional antigens being created. This will also aid the vaccine construct's processing and presentation by SLA to T-cells. To improve the immunogenicity of the vaccine construct, an adjuvant, Sus scrofa β-defensin, was fused to the N-terminal with the aid of an EAAAK linker, which provides rigidity and decreases the likelihood of interference from other protein regions in the adjuvant-receptor interaction. At the C-terminal end, the TAT protein, a cell-penetrating peptide, was appended to the vaccine construct to aid in intracellular delivery. This protein has been harnessed for the delivery of protein-based vaccines, such as ones against Hepatitis B virus40 and Leishmania major41, and invokes enhanced cellular and humoral immune responses. The final vaccine construct, composed of 437 amino acid residues with an instability index score of 34.91, indicates stability, as proteins with an instability index of less than 40 are considered stable. The allergenicity, antigenicity, and stability analyses of the designed vaccine construct showed that it is non-allergic, antigenic, and stable. The 3D structure of the generated vaccine was predicted using Rosetta. To achieve good expression of the vaccine construct in E. coli, codon optimization was employed to enhance transcriptional and translational efficiency. This was attained by analyzing CAI, the total GC content of the DNA sequence, codon frequency distribution, and mRNA stability. The solubility of the vaccine in E. coli is crucial for biochemical and functional investigations. The vaccine construct exhibited justifiable solubility in an overexpressed state. The polar nature and solubility of the vaccine construct, indicated by its low GRAVY index of -0.708, suggest its effective interaction with water42.
The high aliphatic index of 59.70 in the vaccine construct suggests that it may be thermostable. The estimated half-life of the vaccine construct is 1 h (mammalian reticulocytes, in vitro), 30 min (yeast, in vivo), and greater than 10 h (E. coli, in vivo), signifying the time taken by the vaccine construct to reduce to 50% of its initial concentration after production in the cell. Ramachandran plot analysis validated the 3D structure of the vaccine construct, showing 91.8% of residues located in the most-favored regions, 7.0% in additional allowed regions, 0.3% in generously allowed regions, and only 0.8% in disallowed regions. This was further validated by the overall quality factor plot (ERRAT) of the final vaccine construct after refinement processes, which showed a score of 76.1124%. In the ERRAT plot, regions of the 3D model that can be rejected at the 95% confidence level are depicted in grey lines, and regions that can be rejected at the 99% level are shown in black lines. The Z-score of -7.9 revealed that the vaccine construct falls within the plot consisting of Z-scores from already determined structures solved by NMR and X-ray crystallographic experiments, i.e., it is in the range of native protein conformation.
To eliminate ASFV in the system, both innate and adaptive immune systems have to be conscripted. The in silico immune response simulation of the vaccine construct predicted the production of high levels of IFN-γ and a long-lasting cellular response. Furthermore, the C-IMMSIM server predicted the production of IgM + IgG, IgM, IgG1 + IgG2, and IgG1 after immunization with the vaccine construct. These results suggest the likely effectiveness of the vaccine construct against ASF. However, studies have shown that some ASFV proteins, for instance, the I329L protein, inhibit dsRNA-stimulated activation of NF-κB and IRF3, which are two vital players in the innate immune response43. The double-stranded DNA genome of ASFV is expected to be recognized by TLR-9. TLR-9s, type 1 transmembrane endosomal horseshoe-shaped proteins, are expressed on monocytes, macrophages, myeloid dendritic cells, and plasmacytoid DCs44. They interact with PAMPs and signal via MyD88-dependent or TRIF-dependent pathways, resulting in the production of IFNs or pro-inflammatory cytokines. There have been reports of immune evasion mechanisms of ASFV mediated by various genes present in the multigene families MGF360 and 530/505 in virulent ASFV that inhibit type I IFN induction in ASFV-infected macrophages45. However, interferon type II, IFN-γ production, has been reported to be activated by homologous or attenuated isolates of ASFV, but not by stimulation with heterologous or virulent isolates in pigs46. For the adaptive immune response, CTL plays a pivotal role in immune protection against intracellular pathogens, especially viruses47, including ASF. DNA vaccination studies have revealed a correlation between protection from lethal challenge and the presence of a large number of vaccine-induced antigen-specific CD8+ T-cells in vaccinated animals48, 49. Molecular docking revealed detailed interaction between the vaccine construct–TLR-9 and vaccine construct-SLA-1 complexes. The docking interaction between the construct and TLR-9 revealed 14 H-bonds and 5 salt bridges that are involved in the vaccine construct–TLR-9 interaction, and 14 hydrogen bonds and 4 salt bridges formed during the vaccine construct-SLA-1 interaction. Also, in the docked vaccine construct-SLA-1 complex, the interactions are mainly with the PBG pockets A, E, and F, with the major part of the interactions being with pocket F, which shows strong hydrophobic properties. Molecular docking analyses of the vaccine construct-TLR-9 and vaccine construct-SLA-1 complexes indicate very strong interactions in the complexes. Molecular dynamic simulation performed for 10 ns for each complex showed stable interactions of the vaccine construct with the immune receptors, indicating that the immune response can be easily induced. The RMSD plots of each complex revealed stability, and RMSF results showed the vaccine construct had the lowest fluctuations in the regions with the most interactions, especially with SLA-1, which induces cell-mediated immune response. RMSD and RMSF results from this study are in agreement with other studies where the stability and flexibility of proteins were determined. Codon optimization was embarked upon to enhance the expression of the vaccine construct in the E. coli strain K12. The adapted vaccine construct sequence with a GC content of 53.01% is considered reliable since a GC content between 30% and 70% is appropriate for good expression. Furthermore, the vaccine construct was analyzed to determine if it could stimulate the host immune system after interacting with the pig immune receptors in the body. The vaccine construct is expected to induce both cellular and humoral immune responses. With repeated administration of the vaccine construct, there was a general increase in immune response, including an increase in the levels of cytokines IFN-γ, IL-2, IL-10, and IL-12. IFN-γ and IL-2 play roles in the inhibition of viral replication and in the production of T-cells and natural killer cells, respectively, resulting in viral clearance. There was also an increase in IgM+IgG and IgG1+IgG2 levels, which is very important for virus neutralization in the extracellular spaces. Additionally, there was an increase in TH cell levels, which supports both innate and adaptive immune responses as a result of vaccination. A substantial increase in Tc level is expected based on the vaccine construct design, ensuring effective viral clearance from the host system. Furthermore, to achieve good expression of the vaccine construct protein in the E. coli system, codon optimization and mRNA secondary structure analyses were carried out. Thereafter, BamHI and EcoRI restriction sites were introduced at the N-terminal and C-terminal of the optimized sequence, respectively. Then, the sequence with restriction sites was inserted into the pET-28a(+) vector. The expressed vaccine construct showed good solubility based on its polar nature and as reflected by its low GRAVY index from ProtParam analysis. There has been a report on deploying immunoinformatics to develop multi-epitope vaccines against other viral pathogens such as SARS-CoV-2, Yellow Fever virus, Dengue fever virus, etc50. This approach is promising and could be a harbinger for developing safe and highly effective vaccines for all diseases.
CONCLUSION
African swine fever has been ravaging the swine industry in affected countries due to the lack of effective vaccines. This study employed immunoinformatics to develop a multiepitope vaccine against the disease. The vaccine was developed and tested through computational pipelines and was found to be promising. However, experimental studies need to be carried out to validate this claim.
Abbreviations
ABCpred - Antibody B-cell Epitope prediction server, AMBER03 - Assisted Model Building and Energy Refinement 03 (force field), ASF - African Swine Fever, ASFV - African Swine Fever Virus, BamHI - A restriction enzyme, CAI - Codon Adaptation Index, CTL - Cytotoxic T Lymphocyte, E. coli - Escherichia coli, EAAAK — A type of linker used in vaccine design, EcoRI - A restriction enzyme, ExPASy - Expert Protein Analysis System, GPGPG - A type of linker used in vaccine design, GRAVY - Grand Average of Hydropathicity index, GROMACS - GROningen MAchine for Chemical Simulations, HADDOCK - High Ambiguity Driven protein-protein DOCKing, HTL - Helper T Lymphocyte, IEDB - Immune Epitope Database and Analysis Resource, IFN-γ - Interferon Gamma, JCat - Java Codon Adaptation Tool, MGF360 and 530/505 - Multigene Families 360 and 530/505, MHC-II - Major Histocompatibility Complex class II, MyD88 - Myeloid Differentiation primary response gene 88, NF-κB - Nuclear factor kappa-light-chain-enhancer of activated B cells, NMR - Nuclear Magnetic Resonance, PAMPs - Pathogen-Associated Molecular Patterns, pET-28a (+) - A plasmid vector, PDB - Protein Data Bank, PDBsum - A web-based database of summaries and analyses of all PDB structures, pI - Isoelectric Point, PRODIGY - PROtein binDIng enerGY prediction, RAMACHANDRAN plot - A plot to visualize dihedral angles ψ against φ of amino acid residues in protein structure, RMSD - Root Mean Square Deviation, RMSF - Root Mean Square Fluctuation, RNAfold - A web server for RNA secondary structure prediction, Rosetta - A software suite that includes algorithms for computational modeling and analysis of protein structures, SLA - Swine Leukocyte Antigen, SLA-I - Swine Leukocyte Antigen class I, SVMc - Support Vector Machine classifier, TLR-9 - Toll-Like Receptor 9, TRIF - TIR-domain-containing adapter-inducing interferon-β, VaxiJen - A server for prediction of protective antigens, tumor antigens, and subunit vaccines
Acknowledgments
None.
Author’s contributions
Conceptualization and experimental design: OAF; data collection and analysis, COA, OOO, OOA, TAJ; supervision and result interpretation: OAF, COA, BOE; manuscript draft: OOO, OAF, OOA; formal analysis, review and editing: OAF, TAJ, BOE. All authors commented on the previous versions of the manuscript, and read and approved the final manuscript.
Funding
The authors declare that they received no funds, grants, or other support during the preparation of this manuscript.
Availability of data and materials
Data and materials used and/or analyzed during the current study are available from the corresponding author on reasonable request.
Ethics approval and consent to participate
Not applicable.
Consent for publication
Not applicable.
Competing interests
The authors declare that they have no competing interests.
References
-
Montgomery
R.E.,
On
A.,
Form of Swine Fever Occurring in British East Africa (Kenya Colony). The Journal of Comparative Pathology and Therapeutics.
1921;
34
:
159-91
.
View Article Google Scholar -
Odemuyiwa
S.O.,
Adebayo
I.A.,
Ammerlaan
W.,
Ajuwape
A.T.,
Alaka
O.O.,
Oyedele
O.I.,
An outbreak of African Swine Fever in Nigeria: virus isolation and molecular characterization of the VP72 gene of a first isolate from West Africa. Virus Genes.
2000;
20
(2)
:
139-42
.
View Article PubMed Google Scholar -
Luka
P.D.,
Achenbach
J.E.,
Mwiine
F.N.,
Lamien
C.E.,
Shamaki
D.,
Unger
H.,
Genetic Characterization of Circulating African Swine Fever Viruses in Nigeria (2007-2015). Transboundary and Emerging Diseases.
2017;
64
(5)
:
1598-609
.
View Article PubMed Google Scholar -
Iyer
L.M.,
Balaji
S.,
Koonin
E.V.,
Aravind
L.,
Evolutionary genomics of nucleo-cytoplasmic large DNA viruses. Virus Research.
2006;
117
(1)
:
156-84
.
View Article PubMed Google Scholar -
Alejo
A.,
Matamoros
T.,
Guerra
M.,
Andrés
G.,
A Proteomic Atlas of the African Swine Fever Virus Particle. Journal of Virology.
2018;
92
(23)
:
e01293-18
.
View Article PubMed Google Scholar -
Rodríguez
J.M.,
Yáñez
R.J.,
Almazán
F.,
Viñuela
E.,
Rodriguez
J.F.,
African swine fever virus encodes a CD2 homolog responsible for the adhesion of erythrocytes to infected cells. Journal of Virology.
1993;
67
(9)
:
5312-20
.
View Article PubMed Google Scholar -
Doherty
P.C.,
Zinkernagel
R.M.,
H-2 compatibility is required for T-cell-mediated lysis of target cells infected with lymphocytic choriomeningitis virus. The Journal of Experimental Medicine.
1975;
141
(2)
:
502-7
.
View Article PubMed Google Scholar -
Ho
C.S.,
Lunney
J.K.,
Franzo-Romain
M.H.,
Martens
G.W.,
Lee
Y.J.,
Lee
J.H.,
Molecular characterization of swine leucocyte antigen class I genes in outbred pig populations. Animal Genetics.
2009;
40
(4)
:
468-78
.
View Article PubMed Google Scholar -
Ho
C.S.,
Lunney
J.K.,
Lee
J.H.,
Franzo-Romain
M.H.,
Martens
G.W.,
Rowland
R.R.,
Molecular characterization of swine leucocyte antigen class II genes in outbred pig populations. Animal Genetics.
2010;
41
(4)
:
428-32
.
View Article PubMed Google Scholar -
Madden
D.R.,
The three-dimensional structure of peptide-MHC complexes. Annual Review of Immunology.
1995;
13
(1)
:
587-622
.
View Article PubMed Google Scholar -
Doytchinova
I.A.,
Flower
D.R.,
VaxiJen: a server for prediction of protective antigens, tumour antigens and subunit vaccines. BMC Bioinformatics.
2007;
8
(1)
:
4
.
View Article PubMed Google Scholar -
Farrell
D.,
Jones
G.,
Pirson
C.,
Malone
K.,
Rue-Albrecht
K.,
Chubb
A.J.,
Integrated computational prediction and experimental validation identifies promiscuous T cell epitopes in the proteome of Mycobacterium bovis. Microbial Genomics.
2016;
2
(8)
:
e000071
.
View Article PubMed Google Scholar -
Robinson
J.,
Waller
M.J.,
Stoehr
P.,
Marsh
S.G.,
IPD – the Immuno Polymorphism Database. Nucleic acids research.
2005;
41
(Database issue)
:
D1234-40
.
View Article Google Scholar -
Haste Andersen
P.,
Nielsen
M.,
Lund
O.,
Prediction of residues in discontinuous B-cell epitopes using protein 3D structures. Protein Science.
2006;
15
(11)
:
2558-67
.
View Article PubMed Google Scholar -
Gupta
S.,
Kapoor
P.,
Chaudhary
K.,
Gautam
A.,
Kumar
R.,
Raghava
G.P.,
Consortium
undefined Open Source Drug Discovery,
In silico approach for predicting toxicity of peptides and proteins. PLoS One.
2013;
8
(9)
:
e73957
.
View Article PubMed Google Scholar -
Russell
C.D.,
Unger
S.A.,
Walton
M.,
Schwarze
J.,
The human immune response to respiratory syncytial virus infection. Clinical Microbiology Reviews.
2017;
30
(2)
:
481-502
.
View Article PubMed Google Scholar -
Mohan
T.,
Sharma
C.,
Bhat
A.A.,
Rao
D.N.,
Modulation of HIV peptide antigen specific cellular immune response by synthetic α- and β-defensin peptides. Vaccine.
2013;
31
(13)
:
1707-16
.
View Article PubMed Google Scholar -
Spector
C.,
Mele
A.R.,
Wigdahl
B.,
Nonnemacher
M.R.,
Genetic variation and function of the HIV-1 Tat protein. Medical Microbiology and Immunology.
2019;
208
(2)
:
131-69
.
View Article PubMed Google Scholar -
Gasteiger
E.,
Hoogland
C.,
Gattiker
A.,
Duvaud
S.,
Wilkins
M.R.,
Appel
R.D.,
Protein identification and analysis tools on the ExPASy server. Methods in molecular biology.
2005;
1999
(112)
:
531-52
.
View Article Google Scholar -
Saha
S.,
Raghava
G.P.,
Nucleic Acids Res. Alfred: prediction of allergenic proteins and mapping of IgE epitopes. Molecular Immunology.
2006;
34
:
202-9
.
View Article PubMed Google Scholar -
Yang
J.,
Anishchenko
I.,
Park
H.,
Peng
Z.,
Ovchinnikov
S.,
Baker
D.,
Improved protein structure prediction using predicted interresidue orientations. Proceedings of the National Academy of Sciences of the United States of America.
2020;
117
(3)
:
1496-503
.
View Article PubMed Google Scholar -
Xu
D.,
Zhang
Y.,
Improving the physical realism and structural accuracy of protein models by a two-step atomic-level energy minimization. Biophysical Journal.
2011;
101
(10)
:
2525-34
.
View Article PubMed Google Scholar -
Ramachandran
G.N.,
Ramakrishnan
C.,
Sasisekharan
V.,
Stereochemistry of polypeptide chain configurations. Journal of Molecular Biology.
1963;
7
(1)
:
95-9
.
View Article PubMed Google Scholar -
Carty
M.,
Guy
C.,
Bowie
A.G.,
Detection of Viral Infections by Innate Immunity. Biochemical Pharmacology.
2021;
183
:
114316
.
View Article PubMed Google Scholar -
Takeda
K.,
Akira
S.,
Microbial recognition by Toll-like receptors. Journal of Dermatological Science.
2004;
34
(2)
:
73-82
.
View Article PubMed Google Scholar -
Kawai
T.,
Akira
S.,
Innate immune recognition of viral infection. Nature Immunology.
2006;
7
(2)
:
131-7
.
View Article PubMed Google Scholar -
van Zundert
G.C.,
Rodrigues
J.P.,
Trellet
M.,
Schmitz
C.,
Kastritis
P.L.,
Karaca
E.,
The HADDOCK2.2 Web Server: User-Friendly Integrative Modeling of Biomolecular Complexes. Journal of Molecular Biology.
2016;
428
(4)
:
720-5
.
View Article PubMed Google Scholar -
Vangone
A.,
Bonvin
A.M.,
Contacts-based prediction of binding affinity in protein-protein complexes. eLife.
2015;
4
:
e07454
.
View Article PubMed Google Scholar -
Xue
L.C.,
Rodrigues
J.P.,
Kastritis
P.L.,
Bonvin
A.M.,
Vangone
A.,
PRODIGY: a web server for predicting the binding affinity of protein-protein complexes. Bioinformatics (Oxford, England).
2016;
32
(23)
:
3676-8
.
View Article PubMed Google Scholar -
Laskowski
R.A.,
Jab\lońska
J.,
Pravda
L.,
Vařeková
R.S.,
Thornton
J.M.,
PDBsum: structural summaries of PDB entries. Protein Science.
2018;
27
(1)
:
129-34
.
View Article PubMed Google Scholar -
Grote
A.,
Hiller
K.,
Scheer
M.,
Münch
R.,
Nörtemann
B.,
Hempel
D.C.,
JCat: a novel tool to adapt codon usage of a target gene to its potential expression host. Nucleic Acids Research.
2005;
33
(Web Server issue)
:
W526-31
.
View Article PubMed Google Scholar -
Sharp
P.M.,
Li
W.H.,
The codon Adaptation Index- a measure of directional synonymous codon usage bias, and its potential applications. Nucleic Acids Research.
1987;
15
(3)
:
1281-95
.
View Article PubMed Google Scholar -
Rapin
N.,
Lund
O.,
Bernaschi
M.,
Castiglione
F.,
Computational immunology meets bioinformatics: the use of prediction tools for molecular binding in the simulation of the immune system. PLoS One.
2010;
5
(4)
:
e9862
.
View Article PubMed Google Scholar -
Borca
M.V.,
Rai
A.,
Ramirez-Medina
E.,
Silva
E.,
Velazquez-Salinas
L.,
Vuono
E.,
A Cell Culture-Adapted Vaccine Virus against the Current African Swine Fever Virus Pandemic Strain. Journal of Virology.
2021;
95
(14)
:
e0012321
.
View Article PubMed Google Scholar -
Ramirez-Medina
E.,
Vuono
E.,
Rai
A.,
Pruitt
S.,
Espinoza
N.,
Velazquez-Salinas
L.,
Deletion of E184L, a Putative DIVA Target from the Pandemic Strain of African Swine Fever Virus, Produces a Reduction in Virulence and Protection against Virulent Challenge. Journal of Virology.
2022;
96
(1)
:
e0141921
.
View Article PubMed Google Scholar -
Kumar
N.,
Admane
N.,
Kumari
A.,
Sood
D.,
Grover
S.,
Prajapati
V.K.,
Cytotoxic T-lymphocyte elicited vaccine against SARS-CoV-2 employing immunoinformatics framework. Scientific Reports.
2021;
11
(1)
:
7653
.
View Article PubMed Google Scholar -
Dey
J.,
Mahapatra
S.R.,
Patnaik
S.,
Lata
S.,
Kushwaha
G.S.,
Panda
R.K.,
Molecular Characterization and Designing of a Novel Multiepitope Vaccine Construct Against Pseudomonas aeruginosa. International Journal of Peptide Research and Therapeutics.
2022;
28
(2)
:
49
.
View Article PubMed Google Scholar -
Heimerman
M.E.,
Murgia
M.V.,
Wu
P.,
Lowe
A.D.,
Jia
W.,
Rowland
R.R.,
Linear epitopes in African swine fever virus p72 recognized by monoclonal antibodies prepared against baculovirus-expressed antigen. Journal of Veterinary Diagnostic Investigation.
2018;
30
(3)
:
406-12
.
View Article PubMed Google Scholar -
Athanasiou
E.,
Agallou
M.,
Tastsoglou
S.,
Kammona
O.,
Hatzigeorgiou
A.,
Kiparissides
C.,
A poly (lactic-co-glycolic) acid nanovaccine based on chimeric peptides from different Leishmania infantum proteins induces dendritic cells maturation and promotes peptide-specific IFNγ-producing CD8+ T cells essential for the protection against experimental visceral leishmaniasis. Frontiers in Immunology.
2017;
8
:
684
.
View Article PubMed Google Scholar -
Chen
X.,
Lai
J.,
Pan
Q.,
Tang
Z.,
Yu
Y.,
Zang
G.,
The delivery of HBcAg via Tat-PTD enhances specific immune response and inhibits Hepatitis B virus replication in transgenic mice. Vaccine.
2010;
28
(23)
:
3913-9
.
View Article PubMed Google Scholar -
Skwarczynski
M.,
Toth
I.,
Cell-penetrating peptides in vaccine delivery: facts, challenges and perspectives. Therapeutic Delivery.
2019;
10
(8)
:
465-7
.
View Article PubMed Google Scholar -
Kyte
J.,
Doolittle
R.F.,
A simple method for displaying the hydropathic character of a protein. Journal of Molecular Biology.
1982;
157
(1)
:
105-32
.
View Article PubMed Google Scholar -
Wang
T.,
Sun
Y.,
Huang
S.,
Qiu
H.J.,
Multifaceted Immune Responses to African Swine Fever Virus: Implications for Vaccine Development. Veterinary Microbiology.
2020;
249
:
108832
.
View Article PubMed Google Scholar -
Balmelli
C.,
Alves
M.P.,
Steiner
E.,
Zingg
D.,
Peduto
N.,
Ruggli
N.,
Responsiveness of fibrocytes to toll-like receptor danger signals. Immunobiology.
2007;
212
(9-10)
:
693-9
.
View Article PubMed Google Scholar -
Schäfer
A.,
Franzoni
G.,
Netherton
C.L.,
Hartmann
L.,
Blome
S.,
Blohm
U.,
Adaptive Cellular Immunity against African Swine Fever Virus Infections. Pathogens (Basel, Switzerland).
2022;
11
(2)
:
274
.
View Article PubMed Google Scholar -
Alonso
F.,
Domínguez
J.,
Viñuela
E.,
Revilla
Y.,
African swine fever virus-specific cytotoxic T lymphocytes recognize the 32 kDa immediate early protein (vp32). Virus Research.
1997;
49
(2)
:
123-30
.
View Article PubMed Google Scholar -
Barry
M.,
Bleackley
R.C.,
Cytotoxic T lymphocytes: all roads lead to death. Nature Reviews. Immunology.
2002;
2
(6)
:
401-9
.
View Article PubMed Google Scholar -
Argilaguet
J.M.,
Pérez-Martín
E.,
Nofrarías
M.,
Gallardo
C.,
Accensi
F.,
Lacasta
A.,
DNA vaccination partially protects against African swine fever virus lethal challenge in the absence of antibodies. PLoS One.
2012;
7
(9)
:
e40942
.
View Article PubMed Google Scholar -
Takamatsu
H.H.,
Denyer
M.S.,
Lacasta
A.,
Stirling
C.M.,
Argilaguet
J.M.,
Netherton
C.L.,
Cellular immunity in ASFV responses. Virus Research.
2013;
173
(1)
:
110-21
.
View Article PubMed Google Scholar -
Tosta
S.F.,
Passos
M.S.,
Kato
R.,
Salgado
Á.,
Xavier
J.,
Jaiswal
A.K.,
Multi-epitope based vaccine against yellow fever virus applying immunoinformatics approaches. Journal of Biomolecular Structure & Dynamics.
2021;
39
(1)
:
219-35
.
View Article PubMed Google Scholar
Comments
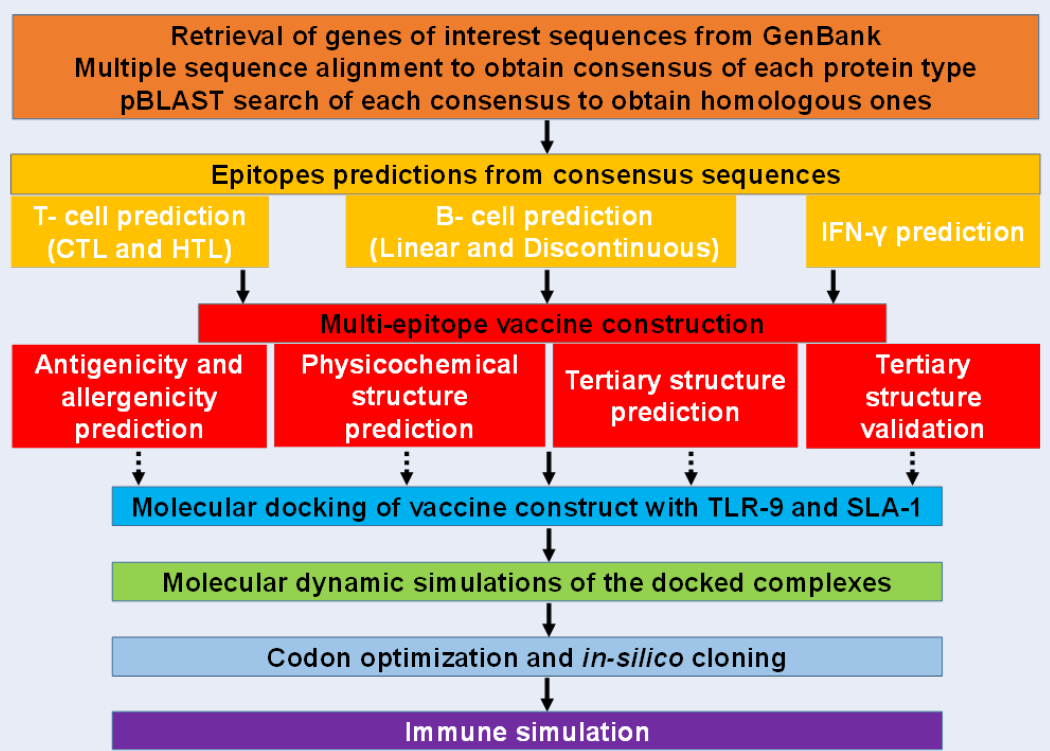
Article Details
Volume & Issue : Vol 11 No 8 (2024)
Page No.: 6642-6660
Published on: 2024-08-31
Citations
Copyrights & License

This work is licensed under a Creative Commons Attribution 4.0 International License.
Search Panel
Pubmed
Google Scholar
Pubmed
Google Scholar
Pubmed
Google Scholar
Pubmed
Google Scholar
Pubmed
Google Scholar
Pubmed
Search for this article in:
Google Scholar
Researchgate
- HTML viewed - 1838 times
- PDF downloaded - 597 times
- XML downloaded - 78 times