Abstract
Heart failure (HF) is considered a leading cause of death in patients with established cardiovascular (CV) and metabolic diseases. Although current treatment strategy has improved survival rate and clinical outcomes of HF, the HF prevalence exhibits growth especially in older patients’ population and survivors after coronary atherothrombotic events. Current clinical guidelines regarding treatment and prevention of HF claim the role of biological markers as pretty easy and powerful tool for diagnosis, risk stratification, and prognostication of HF. However, there is not clear whether all these biological markers are able to equally predict CV death and HF-related outcomes in patients with acute and chronic HF as well as in various phenotypes of HF. The aim of the review is to discuss a role of in risk stratification and individual treatment in patients with different phenotypes of HF.
Introduction
Heart failure (HF) is considered a leading cause of premature cardiovascular (CV) death in patients with established CV disease Ponikowski et al., 2016. Prevalence of HF has been exhibiting a strong tendency to growth worldwide, despite the scientific progress in the field of the two past decades. HF is also characterized by an elevated rate of primary and secondary hospitalization and increased economic burden for patients and their families. Although there are pretty numbers of clinical guidelines, which clearly indicated diagnosis, prevention and evidence-based treatment of HF, a strategy regarding exclusion of HF diagnosis, as well as risk stratification of HF, nature evolution of disease is not well established and requires more development Wettersten and Maisel, 2016. In this context, biological markers reflected several pathophysiological stages of HF have become a powerful and convenient noninvasive tool for diagnosis of HF, a stratification of HF patients at risk of progression, HF severity, and biomarker-guided therapy Ledwidge et al., 2013. The aim of the review is to discuss a role of biomarker-based approaches for much more pretty accurate diagnosis, in-depth risk stratification and individual targeting in treatment amongst patients with HF.
Conventionally used biomarkers of heart failure
Currently updated clinical recommendations have been reported that the natriuretic peptides (NPs), including brain NP (BNP), mid-regional pro-atrial NP (MR-proANP), NT-pro-brain NP (NT-proBNP), mid-regional pro-brain NP (MR-proBNP), galectin-3, high-sensitivity cardiac troponins and soluble suppressor of tumorigenicity-2 (sST2) receptor are the most frequently used biomarkers in routine clinical practice to stratify patients at risk of HF development, a risk of admission / re-admission to the hospital due to HF-related reasons, and a risk of death ( Table 1 ). Most data on cardiac biomarkers have been derived from chronic HF individuals. In contrast, risk prediction in patients admitted with ADHF remains a challenge.
Natriuretic peptides
First NPs were recommended by the European Society of Cardiology and American Heart Association for exclusion HF, and then they were discussed as a tool for risk stratification, and NPs-guided therapy Wettersten and Maisel, 2016. The majority of NPs’ family members (Atrial NP [ANP] and brain [BNP] apart from C-type of NP (CNP) are mechanical stress-related markers. They are actively released by cardiomyocytes as a result in fluid overload, cardiac stretching, as well as due to exposure other causes, i.e. ischemia/necrosis, metabolic and toxic damage, membrane stability loss, and inflammation. In contrast, CNP is secreted from activated endothelial cells and renal cells in response to cytokines’ activation and through endothelium-dependent agonists, i.e. acetylcholine. The biological effects of ANP and BNP are ensured by binding with appropriate NP receptor type A (NPRA). NPRA are expressed at the surfaces of the target cells and are cooperated with cGMP mediating water/electrolytes’ homeostatic effects, i.e. wateruresis/natriuresis, increasing glomerular filtration rate, volume of circulating plasma, as well as suppressing systemic sympathetic activities, maintenance of cardiac output and regulation of blood pressure. Therefore, NPs may ensure anti-proliferative activity and anti-mutagenic effect, mediate vascular dilatation and prevent vascular wall hypertrophy. Additionally, NPs affect modest anti-aldosterone and endothelin-1 effects.
In patients with HF the plasma levels of BNP and NT-proBNP are typically >100pg/ml and >250pg/mL, respectively, while there is high individual biological variability of both biomarkers irrespective presentation of HFrEF or HFpEF. Elevated levels of NPs are well correlated with clinical status and severity of HFrEF/HFpEF patients, a risk of acute HF/acutely decompensated HF (ADHF) regardless etiology of disease, risk of hospital admission / re-admission, as well as all-cause mortality, CV and HF death in individuals with established HF including at discharge period from the hospital after solving HF decompensation. Recently ended STOP-HF (The St Vincent’s Screening to Prevent Heart Failure) trial Ledwidge et al., 2013 was reported that BNP-based screening was able to reduce the composite endpoint of asymptomatic cardiac dysfunction (regardless to systolic or diastolic) with or without newly diagnosed chronic HFrEF/HFpEF that confirms the immense role of screening NPs and early intervention may prevent HF. More recent evidence suggests that NPs along with the next generation of CV biomarkers could provide added predictive value to drug therapy of HF, which could potentially lower HF-related risk of outcomes Chow et al., 2017Yancy et al., 2017.
Biomarkers of myocardial fibrosis
Galectin-3
Galectin-3 is a soluble β-galactoside-binding protein, which is actively secreted by activated mononuclears and macrophages due to inflammatory stimulation. The main biological function of galectin-3 is to activate the fibroblasts for further collagen synthesis Boulogne et al., 2017. Recent pre-clinical and clinical studies revealed the pivotal role of galectin-3 in progressive accumulation of extracellular matrix leading to cardiac fibrosis, cardiac remodeling, and worsening cardiac performances associated with impaired systolic and diastolic function, dilation of cardiac cavities, and induce of cardiac arrhythmias de Freitas Souza et al., 2017. Increased expression of galectin-3 was found in acute HF, ADHF, and chronic HFrEF/HFpEF regardless etiology of disease [8]. Moreover, galectin-3 in exaggerated concentrations was measured in a serum of the patients at risk of HF and CV disease Imran et al., 2017. In patients with acute HF and acutely decompensated HF galectin-3 was associated with NT-proBNP levels and the estimated glomerular filtration rate (GFR) but not with age and serum cardiac troponins Besler et al., 2017. Nowadays galectin-3 is concerned the predictive biomarker for all-cause mortality, CV mortality and HF-related clinical outcomes in patients with established HF. Recent clinical trials have shown that galectin-3 was not superior to NT-proBNP, sST2 receptor, Growth Differentiation Factor (GDF)-15 or high-sensitive C-reactive protein (hsCRP) in prediction of CV mortality and HF death. However the combination of NPs and galectin-3 was much more pretty accurate in predicting HF death compared to either of other biomarkers alone Srivatsan et al., 2015.
Soluble suppressor of tumorigenicity-2 receptor
Soluble suppressor of tumorigenicity-2 receptor (sST2) belongs to the interleukin (IL)-1 receptor family members, which was found in two comprising isoforms, i.e. membrane-bound (ST2L) and soluble (sST2) isoforms. ST2 interplays with its ligand IL-33 and through myocardial mRNA expressions of Th1-related cytokines (tumor necrosis factor-alpha) may directly enhance in cardiac hypertrophy, aggravating fibrosis, cavities dilation with impaired cardiac function. However, the IL-33/ST2 pathway is involved in the pathogenesis of HF across all pathophysiological stages of the disease and regardless its etiology.
sST2 is posed as a cardiac mechanical strain biomarker having useful ability in independent prognostic stratification of HF patients. It has been found that serum levels of sST2 in acute HF / acutely decompensated HF were dramatically increased on admission and appeared to be decreased rapidly depending on clinical improvement. Therefore, sST2 in HF has well correlated with BNP and GDF15 levels Boulogne et al., 2017. Prognostic importance of sST2 was served for prediction of all-cause mortality, CV death and HF admission in HFrEF / HFpEF. However, sST2 levels at discharge were better predictor of HF re-admission than ones at admission. Although both biomarkers of myocardial fibrosis (sST2 receptor and galectin-3) are predictive of HF-related admission to the hospital and CV death Billebeau et al., 2017, direct head-to-head comparison of sST2 and galectin-3 revealed superiority of sST2 over galectin-3 in HF risk stratification Bayes-Genis et al., 2014. However, both biomarkers of fibrosis may provide better incremental prognostic value either NPs’ levels in patients with HF.
Biomarkers of myocardial injury
There are some biomarkers of myocardial injury and necrosis (troponins T and I, myoglobin, heart type of fatty acid binding protein, glutathione transferase P1), which are investigated in details as potential predictors of HF onset and HF-related outcomes Anguita, 2017.
Cardiac troponins
Since last two decades high-sensitivity cardiac troponins (troponins T and I) had been suggested to be prognosticators of higher risk of CV mortality and combined adverse CV outcomes in acutely decompensated or chronic HFrEF/HFpEF Nagarajan et al., 2012. However, cardiac troponins releases from reversibly/irreversibly injured cardiomyocytes and have frequently found in elevated concentrations in patients with acute and chronic HF, there are speculative opinions and controversial evidence regarding their independent relation to acute HF outcomes Masson et al., 2010. Thus, more clinical investigations are required to clear the predictive role of these biomarkers in HF prediction and nature evolution.
Heart type of fatty acid binding protein
The main biological role of heart type of fatty acid binding protein (hFABP) is to facilitate the long-chain fatty acids re-uptake, attenuate calcium transport in cardiomyocytes and regulate inflammatory response in reply to some lipid signals Chmurzyńska, 2006 . hFABP is predominantly expressed in cardiomyocytes and is powerful biomarker of myocardial injury. Recent studies have shown that the hFABP has better predicted CV outcomes to other biomarkers of cardiac damage, i.e. myoglobin and high-sensitive cardiac troponins Qian et al., 2016, whereas elevated intestinal FABP would identify patients with advanced HF who had severe fluid retention and intestinal congestion Kitai et al., 2017. Overall, the hFABP may better provide prognostic information on survival and more precise reflect a risk of major CV events during hospitalization period and short-time after discharge than natriuretic peptides, cardiac troponins and galectin-3. However, the role of several types of FABP in HF is not fully clear. Large clinical studies are required to more accurately explain the predictive value of these biomarkers especially in head-to-head comparison with other molecules, i.e. myoglobin, and glutathione transferase P1.
Biomarker(s)-based strategies of pharmacological and non-pharmacological therapies
Biomarker(s)-guided therapy with serial biomarker values is considered a pretty reliable and as it is suggesting effective method for timely therapeutic adjustment in HF management. Although there are some speculations regarding strong evidence of biomarker-guided HF therapy, the proof-of-concept appears to be promising for individualizing medical care including rehabilitation methods in HF.
Biomarker-based HF-guided therapy
Biomarker(s)-guided therapy with serial biomarker values is considered a pretty reliable and as it is suggesting effective method for timely therapeutic adjustment in HF management. Although there are some speculations regarding strong evidence of biomarker-guided HF therapy, the proof-of-concept appears to be promising for individualizing medical care including rehabilitation methods in HF.
Biomarker-based HF-guided therapy
As it had been suggested the biomarker(s)-guided HF therapy could improve a routine clinical management through adjusted doses / routes of drug(s) and increase a competence regarding decision-making for an admission to the hospital before urgent state onset. Indeed, NP guided HF therapy improves titration of medications. However, taking into consideration the results of recently completed multi center randomized clinical trials there has not obviously become whether biomarker(s)-guided therapy would associate with better HF clinical outcomes during average 6-12 month follow-up Wettersten and Maisel, 2016. Meanwhile, serial measurements biomarkers could be useful for determining severity of HF for interference of ambulatory and in-hospital medical care. Additionally, NT-proBNP, but not BNP, is better suited during HF therapy based on the new angiotensin-receptor-neprilysin-inhibitor (ARNI). Indeed, new era in use of NPs in monitoring of HF evolution has been opened after implementation in the routine clinical practice Malek and Gaikwad, 2017.
Recent clinical trials have been shown that nephrilisin inhibition auxiliary to chronic renin-angiotensin system blockage with LCZ696 (Sacubitril/Valsartan) may increase the bioavailability of NPs and promotes additional benefits the cardio-renal system and hence protected against all-cause mortality, CV mortality and HF death Wong et al., 2017. Because of biologically active BNP is degraded by neprilysin, in HF patients treated with ARNI circulating level of BNP sufficiently increases, whereas NT-proBNP concentration declines dramatically. On this occasion, the principles of NPs-based HF guided therapy are challenging. Apparently, monitoring of BNP levels is not suitable for risk stratification and HF adjusted medical care, when ARNIs are used, however, NT-proBNP remains to be a main key for initiated risk assessment and appraised HF stratification regardless drugs’ prescription Luchner et al., 2017.
There are expectations regarding that the galectin-3- and pro-calcitonin-based HF therapies would be better than NP-guided treatment strategy in HFrEF / HFpEF. However, there is no strong evidence for clinically-proven data about this conception because there are findings for suboptimal sensitivity and/or specificity of HF management Aspromonte et al., 2016.
Biomarker-based cardiac rehabilitation programs
There is a large body of evidence regarding that NT-proBNP, galectin-3, sST2, MR-proADM, and mid-regional pro-adrenomedullin (MR-proANP) could have much more prognostic importance for cardiac rehabilitation programs in HF individuals Billebeau et al., 2017Nakanishi et al., 2017. It has suggested that an overall improvement in the neuro-hormonal profile due to cardiac rehabilitation may correspond to increase of survival probability Nymo et al., 2017, rather in patients with HF with reduced ejection fraction (HFrEF) than in individuals with HF with preserved ejection fraction (HFpEF). Finally, majority of experts believe that a combination of biomarkers may ultimately prove to be more informative in their predictive ability than single biomarker, while this issue is pretty discussable Nymo et al., 2017.
Limitations in use of conventional biomarkers in HF
Confusingly, the role of NPs in modification of treatment care considerably relates to aging, CV disease and metabolic co-morbidities, kidney clearance, metabolism (neprilysin for BNP, glycosylation, methylation, oxidation for other NPs), toxic effect (cardiotoxicity) Berezin, 2016f. Therefore, higher individual biological variability of these biomarkers, which negatively effects on interpretation of measure results Favresse and Gruson, 2017. Additionally, there is a huge list of the diseases associated with increased level of NPs beyond HF development ( Table 2 ).
Although galectin-3 is an independent predictor of all-cause mortality, CV death and occurrence of HF, there is an inverse relationship between serum galectin-3 and estimated glomerular filtration rate Besler et al., 2017. Accordingly, lowered kidney clearance should be taken into consideration, when data of galectin-3 measurement are interpreted. Therefore, older patients contributed to higher galectin-3 concentrations than younger individuals Krintus et al., 2017. Amongst other biomarkers (NPs, GDF-15, high-sensitivity troponin T, sST2, aldosterone, phosphate, parathyroid hormone, plasma renin concentration, and creatinine) galectin-3 had the lowest indices of individual biological variability, whereas NPs and GDF-15 has the highest ones Meijers et al., 2016. Additionally, in contrast to NPs serum galectin-3 levels did not appear to be significantly related to circulating level of cardiac troponins, left ventricular (LV) ejection fraction and LV mass index Agnello et al., 2017. Therefore, there was a positive correlation galectin-3 levels with NT-proBNP in HF individuals. Thus, galectin3 and NPs might be allocated as the best tool for both short- and long term death prediction in HF regardless kidney function and age. Unfortunately, no one biomarker predicted the short-term composite HF endpoints in acute HF and actually decompensated chronic HF Miró et al., 2017. Additionally, there are controversial findings regarding that there was no association of galectin-3 concentration with adverse outcomes in chronic HF Wojciechowska et al., 2017.
Optimistically results of recent clinical trial about higher predictive value of sST2 receptor in HF Maisel and Di Somma, 2016 have associated with some evidence regarding that sST2 was related to increased age, female sex, and some comorbidities including diabetes, atrial fibrillation, inflammatory diseases, kidney insufficiency and myocardial infarction Berezin, 2016b . Additionally, sST2 was not associated with LV structure or LV systolic or diastolic function AbouEzzeddine et al., 2017. Thus, these findings confirmed that the sST2 is rather a systemic inflammatory marker of extra cardiac origin of HF deteriorations than a single prognosticator of HF evolution, while meta-analysis provided by Aimo et al (2017) Aimo et al., 2017 pointed that sST2 level may predict all-cause and CV death in HF patients at admission and at discharge from the hospital. Overall, there is a large body of evidence regarding that improved discriminative value of multiple biomarkers in HF patients requires much more accurate confirmation Berezin et al., 2016bPouleur, 2015. In this context, novel biomarkers are needed to improve in prediction models and assist in the titration of medical therapy.
Novel biomarkers for HF management
The discovery of new biomarkers remains to be promised, but rarely novel molecules prove to be significantly better in diagnostic and predictive manner than established biomarkers. However, additionally to various types of NPs, galectin-3, sST2, and highly sensitive cardiac troponins, multiple other biomarkers, including those of inflammation, oxidative stress, vascular dysfunction and reparation state, biochemical myocardial stress and matrix remodeling, have been implicated in HF management ( Table 3 ). Additionally, there is no strong and clear evidence regarding that the new biomarkers are able to predict clinically significant end-points (i.e., all-cause and CV mortality, HF admission/re-admission, and HF death) in both HF phenotypes - HFpEF and HFrEF. Recent clinical trials have been revealed that majority of new biomarkers indicated rather HF phenotype-related clinical outcomes than independently predicted any end points regardless presentation of HFpEF / HFrEF. Probably, biomarker-based approach could be useful to characterize pathophysiological differences between HFrEF and HFpEF patients.
Inflammatory biomarkers
Creactive protein
The high-sensitive C-reactive protein (hsCRP) is well-established independent predictor for adverse CV outcome including CV death, all-cause mortality, sudden cardiac death, and HF-related death in general population, patients at higher CV risk and amongst individuals with known CV disease Zhu et al., 2017. Recent clinical studies have shown that the levels of hs-CRP were considerably higher in HFpEF than in HFrEF and independently associated with HFrEF development Tromp et al., 2017. Moreover, in HFrEF patients serum hs-CRP levels have positively correlated with circulating NT-proBNP and inversely with left ventricular ejection fraction (LVEF) Kang et al., 2017. In contrast, low to moderate hs-CRP levels did not exhibit an association with HFpFF, while they had to be going to support CV risk.
Calprotectin
Calprotectin (myeloid-related protein 8/14) is an inflammatory marker, which has been found elevated in patients suffering from cardiac conditions, e.g. myocardial infarction, unstable angina and HF. Calprotectin is predominantly expressed in activated human neutrophils, monocytes, adipocytes, and innate immunity cells including macrophages, but not in normal tissue macrophages. Calprotectin binds with Toll-like receptor 4 and acts as innate amplifier of infection, autoimmunity, and cancer. Although calprotectin was found as a nonspecific marker for atherosclerosis, kidney damage, vascular complications in metabolic disease including vascular calcification and endothelial dysfunction, it role in HF is not understood Berezin, 2015a. It was established that patients with chronic HF regardless of LVEF had significantly higher levels of calprotectin than patients without HF Bruhn et al., 2017. However, predictive value of calprotectin requires to be conformed in future.
Procalcitonin
Procalcitonin is known a precursor of the calcitonin, which is produced and actively secreted by the parafollicular C cells of the thyroid gland and involved in regulation of calcium homeostasis Ryu et al., 2015. Recent clinical studies have shown that procalcitonin as an inflammatory biomarker had a pretty accurate diagnostic ability to sepsis, shock, bacterial complications of some diseases Hayashida et al., 2017Morgenthaler et al., 2006Reiner et al., 2017Remde et al., 2016Simon et al., 2004. Additionally, this biomarker may help to manage the patients with HF when antibiotic use is needed or the critical state has been verified Ryu et al., 2015. However, there is not strong evidence regarding procalcitotin use in biomarker-guided therapy to adjust dosage of drugs for HF individuals.
Biomarkers of biochemical myocardial stress
Copeptin
Copeptin is C-terminal peptide derived from the precursor molecule of arginine vasopressin, which plays a pivotal role in fluid retention and electrolyte homeostasis Morgenthaler et al., 2006. In the general population elevated level of copeptin strongly associated with increased CV mortality Remde et al., 2016.
Additionally, based on results of serial measurements of copeptin level it has been suggested that the increased copeptin concentration or trend to elevation of one are an independent risk factor for long-term HF-related clinical outcomes and sudden death in patients with established CV disease Krane et al., 2017Moayedi and Ross, 2017Yan et al., 2017. Being able to better predict all-cause mortality rate and HF-related risks including death and admission to the hospital copeptin might be considered as much more accurate biomarker than NPs for optimize medical care in HF patients Berezin, 2015b. Unfortunately, there are large body of evidence regarding that the level of copeptin might relate closely to some metabolic abnormalities including hyperglycemia that sufficiently limits the predictive power of the biomarker in serial measurements especially in patients with diabetes mellitus and abdominal obesity Savic-Radojevic et al., 2017. However, the improvement of diagnostic reliability of copeptin may achieve by means use of combined biomarker strategy, in particular it might be based on copeptin and NPs Puente et al., 2017Sahin et al., 2016Smaradottir et al., 2017. Finally, circulating level of copeptin has now recognized a promising biomarker with better discriminative value for both all-cause mortality and HF-related outcomes general population and individuals with established CV disease.
Growth differentiation factor-15
Growth differentiation factor (GDF)-15 belongs to the superfamily of transforming growth factor-β Kempf and Wollert, 2009. GDF-15 is widely expressed on the surfaces of various cells. In HF GDF-15 is secreted by injured cardiomyocytes in response to ischemia, reperfusion, inflammatory cytokine stimulation and exposure to biomechanical stress Berezin, 2016d. Elevated level of circulating GDF-15 was found in HF individuals irrespectively etiology of cardiac dysfunction Chan et al., 2016. There is strong evidence regarding being tight interrelationship between circulating level of GDF-15 and HF signs and symptoms, reduced LVEF Hage et al., 2017. Although serial biomarker evaluation has not showed superiority of incremental predictive ability in GDF-15 versus NPs in acute HF Demissei et al., 2017, in chronic HFrHF / HFpEF biomarker strategy based on GDF-15, galectin-3 and NPs might exhibit several advantages before conventional approach in ability to predict all-cause mortality, CV mortality and HF-related outcomes in outpatients with HF Berezin, 2015fBerezin et al., 2015c.
Endothelial cell-derived micro particles and endothelial progenitor cells
Impaired endothelial function plays a pivotal role in the HF development and HF-related complications and also associates with appearance in peripheral blood specific circulating biomarkers, i.e. endothelial micro-particles (EMPs) and endothelial progenitor cells (EPCs) Berezin, 2015fBerezin and Kremzer, 2013cBerezin et al., 2015c. EPCs are involved in the repair of vascular wall and myocardium and, therefore, their ability to restore integrity and functionality of vasculature strongly relates to EMPs. EMPs are not only cargo for cell-to-cell transfer of variety molecules (i.e. peptides, DNAs, RNAs, active molecules, growth factors and hormones), but they are independent regulators of immunity, inflammation, reparation, proliferative response and malignancy Jansen et al., 2017. Number, origin (received from activated cells or apoptotic cells) and immune phenotypes of EMPs can be key factors in ensuring function of endogenous repair system Berezin and Kremzer, 2014b. Thus, EPCs and EMPs are epigenetic co-regulators of vascular function playing a pivotal role in maintenance of endothelium integrity across all stages of HF Berezin, 2016c.
Recent clinical studies have shown that an ability of mature endothelial cells and their precursors to release of secretome progressively worse depended on HF stage and severity Berezin, 2007. Moreover, increased number of apoptotic EMPs and decreased number of EPCs in circulation has found as powerful predictor of CV death, HF-related admission to the hospital and prognosticator of positive reply to medical therapy in short-term period Berezin, 2015cBerezin et al., 2015aBerezin et al., 2014b. There is novel HF risk prediction score created by means of biomarkers including NPs, galectin-3, high sensitive CRP and estimated ratio between both numbers of apoptotic EMPs and EPCs Berezin, 2015c. However, there is not clear whether new predictive model would be effective in discriminative concern of HF treatment Berezin et al., 2015b. More clinical trials are required to improve our understanding in the field of individualized therapy of HF under biomarker control.
Biomarkers of collagen metabolism
Recent studies have shown that impaired collagen metabolism may alter the myocardial collagen network and thereby it exerts cardiovascular remodeling, promotes the fibrotic substrate and mediates HF complications, i.e. atrial fibrillation / flatter, sudden death, and declining LV pump function Löfsjögård et al., 2014. Altered collagen type I synthesis and advance in degradation of one associated with appearance of circulating biomarkers, i.e. carboxy-terminal propeptide (PICP), amino-terminal peptide of procollagen type III (PIIINP) and carboxy-terminal telopeptide (CITP). Interestingly, there is evidence regarding direct causative role of BNP in alterations in collagen type I metabolism in HFrEF. In the OPTIMAL (The Optimizing Congestive Heart Failure Outpatient Clinic trial) was found that disturbances of collagen type I metabolism have exhibited an independent prognostication for long-term all-cause mortality and CV mortality in HFrEF individuals Löfsjögård et al., 2017. In the Cardiovascular Health Study (n=880) in which was included 146 patients with HFrEF (LVEF <55%) 175 patients with HFpEF (LVEF ≥55%), 280 individuals with traditional CV risk factors without chronic HF, and 279 healthy and elderly volunteers with CV disease at risk, biomarkers of collagen turn-over (PIIINP and CITP) were significantly associated with CV outcomes, i.e. death, myocardial infarction, and advanced HF. Therefore, circulating CITP is probably independent predictor of survival in patients with HFrEF. Moreover, CITP level added to NT-proBNP level exhibited an additive predictive value compared with NT-proBNP level alone Tziakas et al., 2012. The estimated negative predictive value for both biomarkers for long-term CV outcomes was 94%. Thus, biomarkers of collagen turn-over might be a powerful component for novel multi-marker strategy for risk stratification of HF.
Biomarkers of cardiovascular remodeling
Matrix metalloproteinase
Adverse cardiac remodeling strong relates to an accumulation of non-fibrillar extracellular matrix (ECM) and matricellular proteins, which contribute to disease progression Berezin and Samura, 2013. In fact, matrix metalloproteinases (MMPs), which are modulated by bio-mechanical and oxidative myocardial stress, neurohormonal and inflammation, essentially determine extracellular reposition of collagen and mediates pro-fibrotic process Sakamuri et al., 2016. According to current understanding in HF the MMPs correspond to an immune activation, inflammation, cardiac injury/vascular dysfunction to maintain tissue structure and metabolism Rienks and Papageorgiou, 2016. MMPs play a pivotal role in cardiac and vascular remodeling through enhancing cell-to-cell interactions acting as regulator of cell growth, proliferation, differentiation, survival, and migration Valiente-Alandi et al., 2016. However, the pathogenetic role of MMPs in HF appears to be uncertain and could relate to etiology of cardiac dysfunction Berezin, 2015b. Resent pre-clinical and clinical studies have shown that impairment of cardiac function may relate to collagen accumulation due to imbalance between expression of MMPs, predominantly MMP-1, MMP-3, MMP-6, MMP-9, and suppression of their tissue inhibitors Berezin et al., 2016cCollier et al., 2011Hutchinson et al., 2010. However, the predictive potency of these biomarkers did not confirm and requires more investigations in future.
Bone-related proteins
Bone-related proteins (BRPs) belong to the family of matricellular proteins, which incorporate into extracellular matrix and play in the bone developing, vascular remodeling, and tissue regeneration Alford and Hankenson, 2006. Amongst family of BRPs osteopontin (OPN), osteoprotegerin (OPG), osteonectin (OSN), osteocalcin (OCN), sclerostin, and some components of RANKL/RANK system are the most known. Overall, all these molecules are mediators for paracrine signaling in cell metabolism and extracellular matrix regulation that relate inflammation with epigenetic regulation of cell function Berezin, 2015e. The role of BRPs in CV disease including HF is controversial. As multiple functional growth factors some members of the BRPs’ family are able to cause cell differentiation and growth, bone and ectopic calcification, vascular remodeling, atherosclerotic plaque shaping, angiogenesis and neovascularisation acting predominantly in result in a hypoxic/ischemia, metabolic, oxidative and inflammatory stimuli Berezin et al., 2016a. On the other hand, BRPs may prevent cardiac dysfunction, hypertrophy and fibrosis through blocking cellular signaling systems (i.e., PI3K and Akt phosphorylation), reduced expression of extracellular matrix and hypertrophy genes Li et al., 2017.
Although OPN, OPG, and RANKL/RANK components are well-known biomarkers of vascular calcification, systemic inflammation, atherosclerosis, kidney dysfunction, and cardiac remodeling, their predictive role in HF is still uncertain Berezin and Kremzer, 2013aBerezin and Kremzer, 2014a. There is evidence regarding that the sRANKL/OPG complex may relate to HFrEF development, whereas circulating levels of OPN and OPG corresponded to HFpEF Berezin and Kremzer, 2014a, c. Moreover, an expression of OPN, OPG and OSN genes in myocardium or vasculature sufficiently distinguished in HFrEF and HFpEF and thereby it has been suggested that BRPs could be markers to suggest the development of different HF phenotypes Cabiati et al., 2016. Indeed, in the PEACE trial levels of OPN were independently associated with the composite CV outcomes and incident HFpEF hospitalization Abdalrhim et al., 2016. Finally, OSN, OPN and OPG have exhibited the predictive value to mortality rate in HFpEF regardless of its etiology, while there was no axillary discriminative effect in entire predictive score when these biomarkers were added to the NPs, hs-CRP, galectin-3 and sST2 Berezin and Kremzer, 2015.
Other biomarkers of vasculogenesis
Although vascular endothelial growth factors (VEGF) act through appropriate receptors A and B, neurophilin may bind some VEGF molecules and contributes in vascular reparation. Thus, both factors are important components of endogenous repair system. Noted, that linear regression followed by network analyses revealed prominent inflammation and angiogenesis-associated interactions through VEGF-related mechanisms in HFpEF and mainly myocardial stretch-associated interactions in HFrEF Berezin, 2015b. The neuropilin has demonstrated a predictive value for all-cause mortality and HF-related re-admission at 18 months in HFpEF, but not in HFrEF. Overall, the role of VEGF-related biomarkers in prediction of HF is not clear and needs to be explained in details in future.
Biomarkers of oxidative stress
Serum uric acid
Observational and clinical studies have shown that the elevated serum uric acid (SUA) is common feature for patients with CV disease including HF, hypertension, atherosclerosis, obesity, diabetes mellitus and chronic renal disease Borghi et al., 2015Grassi et al., 2013. Evidence regarding the role of SUA in pathogenesis of CV disease is controversial. On the one hand, SUA attenuates oxidative stress through overproduction of reactive oxygen species and consequently it often worse vascular / endothelial function indirectly via inflammatory damage, inducing vascular calcification and directly via cell membranes deterioration effect Berezin and Kremzer, 2013b. On the other hand, low-grading inflammation that is frequently found in HF may cause xanthine oxidase over-activity and leads to increased tissue SUA accumulation, which acts as scavenger of free radicals and protects against an damage effect of oxidative stress Berezin, 2014. Additionally, an increase of SUA may be an attribute of lowered kidney clearance as a result in a progress of HF. Therefore, there is evidence regarding the regulatory role of SUA in EPC mobbing and differentiation that allow discussing SUA as mediator of reparation of tissue in HF Berezin et al., 2014a.
Numerous clinical studies have emphasized the predictive role of baseline SUA for early post-discharge HF outcomes Amin et al., 2017Okazaki et al., 2016. Although levels of SUA did not significantly changed for admission period in HF patients, SUA at admission could be considered as powerful prognosticator of ADHF Okazaki et al., 2017. On the other hand, an elevated SUA level on admission in patients with acute HF or ADHF associated not only with HF severity, but with the presence of chronic renal disease and the use of loop diuretics, which are able to cause negative clinical outcomes and independent predictor of 1-year mortality through elevation of SUA Okazaki et al., 2017Okazaki et al., 2016. Interestingly, the activity of xanthine oxidoreductase that is a key rate-limiting enzyme of purine degradation may be more accurate predictor of HFrEF severity and HF clinical outcomes than SUA Otaki et al., 2017. Consequently, SUA remains a well-known risk factor of HF related clinical outcomes in acute HF and ADHF, while poor prognosis in patients with both phenotypes of chronic HF (HFrEF and HFpEF) is not elucidated.
Other biomarkers
Serum levels of myeloperoxidase, ceruloplasmin and 8-hydroxy-2'-deoxyguanosine closely correlated with stage of chronic HF regardless LVEF and predict a development of HFrEF, while the role of these biomarkers of oxidative stress remains under scientific discussion and requires more investigations Berezin, 2015b.
Biomarkers of renal dysfunction
Cystatin C
Cystatin C is an endogenous inhibitor of cysteine proteases and this biomarker is widely discussed an alternative predictor of CV events in acute and chronic HF patients with any types of cardiorenal syndrome. The patients with HFrEF demonstrated elevated serum cystatin C, especially in cases with serious risk of CV complications. Additionally, in hypertensive patients with HFpEF increased cystatin C level was found Huerta et al., 2016. Therefore, it associated with LV diastolic dysfunction and alterations in collagen metabolism regardless of estimated GFR [106]. Although cystatin C has now validated a powerful predictor of CV outcomes and kidney injury, its sensitivity in patients with chronic HF is sufficiently inferior to that of hs-CRP and NPs Berezin, 2015b. In contrast, in acute HF and ADHF Cystatin C provided an incremental value for prognosis more than NT-proBNP and SUA Kim et al., 2013Kim et al., 2015.
Other biomarkers of kidney injury in HF
There is a large body of perspective biomarkers of kidney injury that could be useful for stratification of HF at risk, i.e. stromal cell-derived factor-1, exosomes, MPs, neutrophil gelatinase-associated lipocalin, kidney injury molecule-1, interleukin-18 and miRNAs Taub et al., 2012. Although they are able to emerge at the early stages of renal dysfunction prior to any elevations in serum creatinine, the prognostication of clinical outcomes due to acute HF, ADHF and chronic HF require more investigation.
Genetic biomarkers
By now, genetic testing has incorporated as a part of patient evaluation for suspected inherited cardiomyopathies Teekakirikul et al., 2013Teo et al., 2015. It turns out the epigenetic modifications through DNA methylation, ATP-dependent chromatin remodeling, histone modifications with an involvement of microRNA-related mechanisms might be sufficient pathophysiological factors contributing to adverse cardiac remodeling and altered cardiac function Hershberger and Siegfried, 2011. In this context, the novel risk scores reflecting variabilities in genetic and epigenetic features in HF development appear to be promised Berezin, 2016cLopes and Elliott, 2013Yang et al., 2015. Indeed, some early studies have reported interested results with respect to genetic precursors of HFpEF and HFrEF Berezin, 2016dFazakas et al., 2016Friedrich et al., 2013Hofman et al., 2010Kolder et al., 2012McNamara et al., 2014Sutter et al., 2013. As biomarkers particularly used to scrutiny single nucleotide polymorphisms (SNPs) of genes encoding enzymes related to oxidative stress Berezin, 2016e, genotype of guanine nucleotide-binding proteins (G-proteins) beta-3 subunit (GNB3) Fazakas et al., 2016, transcription factor Islet-1 gene McNamara et al., 2014, troponin T Friedrich et al., 2013, CYP2D6 polymorphism Hofman et al., 2010, cardiac myosin binding protein-C mutations Friedrich et al., 2013, renin-angiotensin-aldosterone system polymorphism Sutter et al., 2013 etc. Indeed, it is well known that angiotensin-converting enzyme (ACE) I/D gene D allele was associated with higher overall mortality as compared with the I allele in HF patients and that the effect could be modified by ACE inhibitors’ given Kolder et al., 2012. Additionally, ACE DD and angiotensin-1-receptor 1166 CC genotypes may synergistically increase the predisposition to HFpEF Wu et al., 2010.
Unfortunately, in ARIC (Atherosclerosis Risk in Communities) study was reported that none of the metabolite SNPs including pyroglutamine, dihydroxy docosatrienoic acid were individually associated with incident HF, whereas a genetic risk score created by summing the most sufficient risk alleles from each metabolite determined 11% greater risk of HF per allele Wu et al., 2009. Ganna et al (2013) Yu et al., 2013 have reported that amongst 707 common SNPs associated with 125 diseases including HF it would not be easily obtained explainable results by common genetic variants related to HF development. Consequently, a close gene-gene interaction may determine an individual risk to development of HF through different pathways including epigenetic modifications. All these findings lead to assume that genes score might be a powerful tool for prediction of HF development.
More successful genome-wide linkage studies toward genes-related contribution in HF have been devoted incorporating SNPs of several genes (i.e. the bradykinin type 1 receptor gene, angiotensin-II type I receptor gene, the β1-adrenoceptor gene and CYP2D6 polymorphysm) in predictive score to benefit and suffer harm from HF therapy. Although these parmacogenetic studies have focused on promised topics, the obtained results have not been absolutely consistent Bondar et al., 2014Ganna et al., 2013Nelveg-Kristensen et al., 2015Yip and Pirmohamed, 2013. Nelveg-Kristensen et al (2015) Yip and Pirmohamed, 2013 have found no sufficient association between pharmacogenetic scores and fatal outcomes in HF patients. In contrast, Bondar et al (2014) Nelveg-Kristensen et al., 2015 have guessed that the gene expression profiling might be useful rather for risk prediction in HF than for choosing HF treatment regime. Thus, the clinical implementation of the HF therapy based on genes scoring remains uncertain and requires more evaluation in the future Berezin, 2016a.
Multiple biomarker predictive scores
Multiple biomarkers’ use strategies based on the combination of NPs with other biomarkers have been discussed as priority in creating of much more accurate predictive scores in HF Bayes-Genis and Ordonez-Llanos, 2015. Although there are several predictive scores based on biomarker measurement and approved for chronic HF, predictive scores for and acutely decompensated HF have not been validated Cohen-Solal et al., 2015. Current multiple biomarker score toward prognostication, risk stratification and diagnosis of HF is based on NPs in combination with biomarkers of myocardial injury and fibrosis (galectin-3 and sST2 receptor). It is validated by American Heart Association / American College of Cardiology at 2017 and the score is suitable for patients at risk of HF, individuals with established chronic HF (for both HFrEF and HFpEF), patients with suspected acute HF and documented acute / acutely decompensated HF, as well as patients with HF at discharge from the hospital. However, there is need to compare novel scores with recently created and the scores used in HFrEF and HFpEF to optimize the treatment approach in HF management Berezin, 2015d.
Recently developed biomarkers, i.e. mid-regional pro-A-type natriuretic peptide (Mid Pro-ANP), mid-regional-proadrenomedullin (MR-proADM), pro-endothelin, and copeptin, when were added to the predictive model based on well-known prognostic biomarkers (NPs, troponin, hs-CRP, pro-calcitonin), have been investigated in 28-days predictive value of entire score in patients with severe acute dyspnea and suspecting to acute HF or ADHF. Although three biomarkers Mid Pro-ANP, MR-proADM and pro-endothelin - have been independently associated with prognosis of acute and chronic HF regardless LFEF, MR-proADM had improved discriminative value of NPs in combination with copeptin and troponin T Ara-Somohano et al., 2017. Overall, there is no clarity and consistent evidence for multiple biomarker strategy in improvement in CV mortality and CV outcomes. It has been suggesting that sST2, MR-proADM and galectin-3 could improve prognostication of HF-related hospitalization and death, when they are added to NPs.
Conclusions
There are several controversies regarding importance of predictive value for survival and incremental prognostication in diagnosis of HFrEF and HFpEF. Probably, biomarkers of inflammation and vascular remodeling are predominantly observed in HFpEF, while biomarkers of biomechanical stress and collagen metabolism much more accurately predicted clinical outcome in HFrEF. All these require improving clinical guideline recommendations for optimizing HF therapy in routine clinical practice under biomarkers’ control. There is need in larger clinical trials to head-to-head compare different biomarkers and clear their role in diagnosis and guided therapy of HF.
Abbreviations
ADM: adrenomedullin
ANP: atrial natriuretic peptide
ARNI: angiotensin receptor neprilysin inhibitors
BNP: brain natriuretic peptide
BRPs: Bone-related proteins
cGMP: cyclic guanylyl monophosphate
CITP: carboxy-terminal telopeptide
CNP: C-type natriuretic peptide
CRP: C-reactive protein
CT-proET-1: C-terminal-pro-endothelin-1
CV: cardiovascular
EMPs: endothelial microparticles
EPCs: endothelial progenitor cells
Gal-3: galectin-3
GDF-15: Growth differentiation factor-15
GFR: glomerular filtration rate
HF: heart failure
hFABP: heart type of fatty acid binding protein
HFpEF: heart failure with preserved ejection fraction
HFrEF: heart failure with reduced ejection fraction
LV: left ventricular
MMP: matrix metalloproteinase
MPs: micro particles
MR: proANP – mid-regional pro-atrial natriuretic peptide
MR: proADM - mid-regional pro-adrenomedullin
NPs: natriuretic peptides
OCN: osteocalcin
OPG: osteoprotegerin
OPN: osteopontin
OSN: osteonectin
PICP: carboxy-terminal propeptide
RANKL: receptor activator of nuclear factor-κB ligand
sST2: soluble suppressor of tumorigenicity-2 receptor
SUA: serum uric acid
References
-
A.D.
Abdalrhim,
T.S.
Marroush,
E.E.
Austin,
B.J.
Gersh,
N.
Solak,
S.A.
Rizvi,
K.R.
Bailey,
I.J.
Kullo.
Plasma Osteopontin Levels and Adverse Cardiovascular Outcomes in the PEACE Trial. PloS one.
2016;
11
:
e0156965
.
-
O.F.
AbouEzzeddine,
P.M.
McKie,
S.M.
Dunlay,
S.R.
Stevens,
G.M.
Felker,
B.A.
Borlaug,
H.H.
Chen,
R.P.
Tracy,
E.
Braunwald,
M.M.
Redfield.
Suppression of Tumorigenicity 2 in Heart Failure With Preserved Ejection Fraction. Journal of the American Heart Association.
2017;
6
:
e004382
.
-
L.
Agnello,
G.
Bivona,
B.L.
Sasso,
C.
Scazzone,
V.
Bazan,
C.
Bellia,
M.
Ciaccio.
Galectin-3 in acute coronary syndrome. Clinical Biochemistry.
2017;
S0009-9120
:
30245-X
.
-
A.
Aimo,
G.
Vergaro,
A.
Ripoli,
A.
Bayes-Genis,
D.A.P.
Figal,
R.A.
de Boer,
J.
Lassus,
A.
Mebazaa,
E.
Gayat,
T.
Breidthardt.
Meta-Analysis of Soluble Suppression of Tumorigenicity-2 and Prognosis in Acute Heart Failure. JACC: Heart Failure.
2017;
5
:
287-296
.
-
A.I.
Alford,
K.D.
Hankenson.
Matricellular proteins: extracellular modulators of bone development, remodeling, and regeneration. Bone.
2006;
38
:
749-757
.
-
A.
Amin,
M.
Chitsazan,
F.
Shiukhi Ahmad Abad,
S.
Taghavi,
N.
Naderi.
On admission serum sodium and uric acid levels predict 30 day rehospitalization or death in patients with acute decompensated heart failure. ESC Heart Failure.
2017;
4
:
162-168
.
-
M.
Anguita.
High-sensitivity troponins and prognosis of heart failure. Revista clinica espanola.
2017;
217
:
95-96
.
-
C.
Ara-Somohano,
A.
Bonadona,
F.
Carpentier,
P.
Pavese,
A.
Vesin,
R.
Hamidfar-Roy,
C.
Minet,
G.
Vanzetto,
C.
Schwebel,
J.
Timsit.
Evaluation of eight biomarkers to predict short term mortality in patients with acute severe dyspnea. Minerva anestesiologica 10.23736/S20375-29393.
2017;
23717
:
10882-23735
.
-
N.
Aspromonte,
M.
Gulizia,
A.
Clerico,
G.
Di Tano,
M.
Emdin,
M.
Feola,
M.
Iacoviello,
R.
Latini,
A.
Mortara,
R.
Valle.
ANMCO/ELAS/SIBioC Consensus document: Recommendations for the use of cardiac biomarkers in heart failure patients. Giornale italiano di cardiologia (2006).
2016;
17
:
615
.
-
A.
Bayes-Genis,
M.
de Antonio,
J.
Vila,
J.
Peñafiel,
A.
Galán,
J.
Barallat,
E.
Zamora,
A.
Urrutia,
J.
Lupón.
Head-to-head comparison of 2 myocardial fibrosis biomarkers for long-term heart failure risk stratification: ST2 versus galectin-3. Journal of the American College of Cardiology.
2014;
63
:
158-166
.
-
A.
Bayes-Genis,
J.
Ordonez-Llanos.
Multiple biomarker strategies for risk stratification in heart failure. Clinica Chimica Acta.
2015;
443
:
120-125
.
-
Berezin.
The Myeloid-Related Protein Complex Calprotectin as Biomarker of Cardiovascular Risk in Diabetes Mellitus Patients. Diabetes Res Treat.
2015a;
:
129-136
.
-
Berezin.
Part 4. Diagnostic and prognostic value of biological markers at risk stratification among patients with heart failure. Moskow: LAMBERT Academic Publishing GmbH.
2015b
.
-
Berezin.
Genetic Predictive Scores in Heart Failure: Possibilities and Expectations. J Data Mining Genomics Proteomics.
2016a;
7
:
e127-e128
.
-
A.
Berezin.
Serum uric acid as a metabolic regulator of endothelial reparative processes in heart failure patients. Stem Cell and Translational Investigation.
2014;
1
:
e432
.
-
A.
Berezin.
Impaired pattern of endothelial derived microparticles in heart failure patients. J Mol Genet Med.
2015c;
9
:
2380-0682
.
-
A.
Berezin.
Biomarkers for cardiovascular risk in diabetic patients. Heart.
2016b;
102
:
1939-1941
.
-
A.
Berezin.
Epigenetics in heart failure phenotypes. BBA Clin.
2016c;
6
:
31-37
.
-
A.
Berezin,
A.
Kremzer.
Circulating osteopontin as a marker of early coronary vascular calcification in type two diabetes mellitus patients with known asymptomatic coronary artery disease. Atherosclerosis.
2013a;
229
:
475-481
.
-
A.
Berezin,
A.
Kremzer.
Serum uric Acid as a marker of coronary calcification in patients with asymptomatic coronary artery disease with preserved left ventricular pump function. Cardiology research and practice.
2013b;
2013
:
129369
.
-
A.E.
Berezin.
Endothelial derived micro particles: biomarkers for heart failure diagnosis and management. Br J Haematol.
2007;
137
:
36-48
.
-
A.E.
Berezin.
Biomarker-Guided Therapy for Chronic Heart Failure. In Biomarkers in Cardiovascular Disease, V.B. Patel, and V.R. Preedy, eds.. Dordrecht: Springer Netherlands.
2015d;
:
1-21
.
-
A.E.
Berezin.
Bone-Related Proteins as Markers in Vascular Remodeling. In Biomarkers in Bone Disease, V.R. Preedy, ed. (Springer).
2015e;
:
1-22
.
-
A.E.
Berezin.
The risk stratification in heart failure patients: The controversial role of high-sensitive ST2. inflammation.
2015f;
411
:
20-27
.
-
A.E.
Berezin.
Diabetes mellitus related biomarker: The predictive role of growth-differentiation factor-15. Diabetes & Metabolic Syndrome: Clinical Research & Reviews.
2016d;
10
:
S154-S157
.
-
A.E.
Berezin.
Epigenetically Modified Endothelial Progenitor Cells in Heart Failure. Journal of Clinical Epigenetics.
2016e;
2
:
13
.
-
A.E.
Berezin.
Prognostication in different heart failure phenotypes: the role of circulating biomarkers. Journal of Circulating Biomarkers.
2016f;
5
:
6
.
-
A.E.
Berezin,
A.A.
Kremzer.
Analysis of various subsets of circulating mononuclear cells in asymptomatic coronary artery disease. Journal of clinical medicine.
2013c;
2
:
32-44
.
-
A.E.
Berezin,
A.A.
Kremzer.
Predictive Value of Circulating SPARC-Related protein Osteonectin in Patients with Symptomatic Moderate-to-Severe Ischemic-Induced Chronic Heart Failure. International Journal of Cardiology and Lipidology Research.
2014a;
1
:
43-51
.
-
A.E.
Berezin,
A.A.
Kremzer.
Relationship between circulating endothelial progenitor cells and insulin resistance in non-diabetic patients with ischemic chronic heart failure. Diabetes & Metabolic Syndrome: Clinical Research & Reviews.
2014b;
8
:
138-144
.
-
A.E.
Berezin,
A.A.
Kremzer.
Relationship between serum RANKL/ osteoprotegerin complex and endothelial progenitor cells in ischemic chronic heart failure. Journal of Cardiology and Therapy.
2014c;
1
:
189-195
.
-
A.E.
Berezin,
A.A.
Kremzer.
Predictive value of circulating osteonectin in patients with ischemic symptomatic chronic heart failure. Biomedical Journal.
2015;
38
:
523-530
.
-
A.E.
Berezin,
A.A.
Kremzer,
T.A.
Berezina,
Y.V.
Martovitskaya.
Pattern of circulating microparticles in chronic heart failure patients with metabolic syndrome: Relevance to neurohumoral and inflammatory activation. BBA clinical.
2015a;
4
:
69-75
.
-
A.E.
Berezin,
A.A.
Kremzer,
T.A.
Berezina,
Y.V.
Martovitskaya,
E.A.
Gromenko.
Relation of osteoprotegerin level and numerous of circulating progenitor mononuclears in patients with metabolic syndrome. Biomedical Research and Therapy.
2016a;
3
:
501-513
.
-
A.E.
Berezin,
A.A.
Kremzer,
Y.V.
Martovitskaya,
T.A.
Berezina,
T.A.
Samura.
The utility of biomarker risk prediction score in patients with chronic heart failure. Clinical hypertension.
2016b;
22
:
3
.
-
A.E.
Berezin,
A.A.
Kremzer,
Y.V.
Martovitskaya,
T.A.
Samura,
T.A.
Berezina.
The predictive role of circulating microparticles in patients with chronic heart failure. BBA clinical.
2015b;
3
:
18-24
.
-
A.E.
Berezin,
A.A.
Kremzer,
Y.V.
Martovitskaya,
T.A.
Samura,
T.A.
Berezina,
A.
Zulli,
J.
Klimas,
P.
Kruzliak.
The utility of biomarker risk prediction score in patients with chronic heart failure. International journal of clinical and experimental medicine.
2015c;
8
:
18255-18264
.
-
A.E.
Berezin,
A.A.
Kremzer,
T.A.
Samura.
Circulating thrombospondin-2 in patients with moderate-to-severe chronic heart failure due to coronary artery disease. Journal of biomedical research.
2016c;
30
:
32
.
-
A.E.
Berezin,
A.A.
Kremzer,
T.A.
Samura,
T.A.
Berezina,
Y.V.
Martovitskaya.
Serum uric Acid predicts declining of circulating proangiogenic mononuclear progenitor cells in chronic heart failure patients. Journal of cardiovascular and thoracic research.
2014a;
6
:
153
.
-
A.E.
Berezin,
A.A.
Kremzer,
T.A.
Samura,
Y.V.
Martovitskaya.
Apoptotic microparticles to progenitor mononuclear cells ratio in heart failure: relevance of clinical status and outcomes. JCvD.
2014b;
2
:
50-57
.
-
A.E.
Berezin,
T.A.
Samura.
Prognostic value of biological markers in myocardial infarction patients. Asian Cardiovascular and Thoracic Annals.
2013;
21
:
142-150
.
-
C.
Besler,
D.
Lang,
D.
Urban,
K.-P.
Rommel,
M.
von Roeder,
K.
Fengler,
S.
Blazek,
R.
Kandolf,
K.
Klingel,
H.
Thiele.
Plasma and Cardiac Galectin-3 in Patients With Heart Failure Reflects Both Inflammation and Fibrosis. Circulation: Heart Failure.
2017;
10
:
e003804
.
-
G.
Billebeau,
N.
Vodovar,
M.
Sadoune,
J.M.
Launay,
F.
Beauvais,
A.
Cohen-Solal.
Effects of a cardiac rehabilitation programme on plasma cardiac biomarkers in patients with chronic heart failure. Eur J Prev Cardiol.
2017;
:
2047487317705488
.
-
G.
Bondar,
M.
Cadeiras,
N.
Wisniewski,
J.
Maque,
J.
Chittoor,
E.
Chang,
M.
Bakir,
C.
Starling,
K.
Shahzad,
P.
Ping.
Comparison of whole blood and peripheral blood mononuclear cell gene expression for evaluation of the perioperative inflammatory response in patients with advanced heart failure. PloS one.
2014;
9
:
e115097
.
-
C.
Borghi,
E.A.
Rosei,
T.
Bardin,
J.
Dawson,
A.
Dominiczak,
J.T.
Kielstein,
A.J.
Manolis,
F.
Perez-Ruiz,
G.
Mancia.
Serum uric acid and the risk of cardiovascular and renal disease. Journal of hypertension.
2015;
33
:
1729-1741
.
-
M.
Boulogne,
M.
Sadoune,
J.
Launay,
M.
Baudet,
A.
Cohen-Solal,
D.
Logeart.
Inflammation versus mechanical stretch biomarkers over time in acutely decompensated heart failure with reduced ejection fraction. International Journal of Cardiology.
2017;
226
:
53-59
.
-
L.V.
Bruhn,
K.G.
Lauridsen,
A.S.
Schmidt,
H.
Rickers,
L.F.
Bach,
B.
Løfgren,
N.
Hornung.
Elevated calprotectin in patients with atrial fibrillation with and without heart failure. Scandinavian Journal of Clinical and Laboratory Investigation.
2017;
77
:
210-215
.
-
M.
Cabiati,
B.
Svezia,
M.
Matteucci,
L.
Botta,
A.
Pucci,
M.
Rinaldi,
C.
Caselli,
V.
Lionetti,
S.
Del Ry.
Myocardial Expression Analysis of Osteopontin and Its Splice Variants in Patients Affected by End-Stage Idiopathic or Ischemic Dilated Cardiomyopathy. PloS one.
2016;
11
:
e0160110
.
-
M.M.
Chan,
R.
Santhanakrishnan,
J.P.
Chong,
Z.
Chen,
B.C.
Tai,
O.W.
Liew,
T.P.
Ng,
L.H.
Ling,
D.
Sim,
K.T.G.
Leong.
Growth differentiation factor 15 in heart failure with preserved vs. reduced ejection fraction. European journal of heart failure.
2016;
18
:
81-88
.
-
A.
Chmurzyńska.
The multigene family of fatty acid-binding proteins (FABPs): function, structure and polymorphism. Journal of applied genetics.
2006;
47
:
39-48
.
-
S.L.
Chow,
A.S.
Maisel,
I.
Anand,
B.
Bozkurt,
R.A.
de Boer,
G.M.
Felker,
G.C.
Fonarow,
B.
Greenberg,
J.L. Jr.
Januzzi,
M.S.
Kiernan.
Role of Biomarkers for the Prevention, Assessment, and Management of Heart Failure: A Scientific Statement From the American Heart Association. Circulation.
2017;
135
:
e1054-e1091
.
-
A.
Cohen-Solal,
S.
Laribi,
S.
Ishihara,
G.
Vergaro,
M.
Baudet,
D.
Logeart,
A.
Mebazaa,
E.
Gayat,
N.
Vodovar,
D.A.
Pascual-Figal.
Prognostic markers of acute decompensated heart failure: the emerging roles of cardiac biomarkers and prognostic scores. Archives of cardiovascular diseases.
2015;
108
:
64-74
.
-
P.
Collier,
C.J.
Watson,
V.
Voon,
D.
Phelan,
A.
Jan,
G.
Mak,
R.
Martos,
J.A.
Baugh,
M.T.
Ledwidge,
K.M.
McDonald.
Can emerging biomarkers of myocardial remodelling identify asymptomatic hypertensive patients at risk for diastolic dysfunction and diastolic heart failure?. European journal of heart failure.
2011;
13
:
1087-1095
.
-
B.S.
Freitas Souza,
D.N.
Silva,
R.H.
Carvalho,
G.L.
de Almeida Sampaio,
B.D.
Paredes,
L.A.
França,
C.M.
Azevedo,
J.F.
Vasconcelos,
C.S.
Meira,
P.C.
Neto.
Association of Cardiac Galectin-3 Expression, Myocarditis, and Fibrosis in Chronic Chagas Disease Cardiomyopathy. The American Journal of Pathology.
2017;
187
:
1134-1146
.
-
B.G.
Demissei,
G.
Cotter,
M.F.
Prescott,
G.M.
Felker,
G.
Filippatos,
B.H.
Greenberg,
P.S.
Pang,
P.
Ponikowski,
T.M.
Severin,
Y.
Wang.
A multimarker multi-time point-based risk stratification strategy in acute heart failure: results from the RELAX-AHF trial. European Journal of Heart Failure.
2017;
10.1002/ejhf.1749
.
-
J.
Favresse,
D.
Gruson.
Natriuretic peptides: degradation, circulating forms, dosages and new therapeutic approaches. Paper presented at: Annales de Biologie Clinique.
2017
.
-
Á.
Fazakas,
Z.
Szelényi,
G.
Szénási,
G.
Nyírő,
P.M.
Szabó,
A.
Patócs,
N.
Tegze,
B.C.
Fekete,
A.
Molvarec,
B.
Nagy.
Genetic predisposition in patients with hypertension and normal ejection fraction to oxidative stress. Journal of the American Society of Hypertension.
2016;
10
:
124-132
.
-
F.W.
Friedrich,
G.
Dilanian,
P.
Khattar,
D.
Juhr,
L.
Gueneau,
P.
Charron,
V.
Fressart,
J.T.
Vilquin,
R.
Isnard,
L.
Gouya.
A novel genetic variant in the transcription factor Islet-1 exerts gain of function on myocyte enhancer factor 2C promoter activity. European journal of heart failure.
2013;
15
:
267-276
.
-
A.
Ganna,
F.
Rivadeneira,
A.
Hofman,
A.G.
Uitterlinden,
P.K.
Magnusson,
N.L.
Pedersen,
E.
Ingelsson,
H.
Tiemeier.
Genetic determinants of mortality. Can findings from genome-wide association studies explain variation in human mortality? Human genetics.
2013;
132
:
553-561
.
-
D.
Grassi,
L.
Ferri,
G.
Desideri,
P.
Di Giosia,
P.
Cheli,
R.
Del Pinto,
G.
Properzi,
C.
Ferri.
Chronic hyperuricemia, uric acid deposit and cardiovascular risk. Current pharmaceutical design.
2013;
19
:
2432-2438
.
-
C.
Hage,
E.
Michaëlsson,
C.
Linde,
E.
Donal,
J.-C.
Daubert,
L.-M.
Gan,
L.H.
Lund.
Inflammatory Biomarkers Predict Heart Failure Severity and Prognosis in Patients With Heart Failure With Preserved Ejection Fraction. Circulation: Cardiovascular Genetics.
2017;
10
:
e001633
.
-
K.
Hayashida,
Y.
Kondo,
Y.
Hara,
M.
Aihara,
K.
Yamakawa.
Head-to-head comparison of procalcitonin and presepsin for the diagnosis of sepsis in critically ill adult patients: a protocol for a systematic review and meta-analysis. BMJ open.
2017;
7
:
e014305
.
-
R.E.
Hershberger,
J.D.
Siegfried.
Update 2011: clinical and genetic issues in familial dilated cardiomyopathy. J Am Coll Cardiol.
2011;
57
:
1641-1649
.
-
N.
Hofman,
I.
van Langen,
A.A.
Wilde.
Genetic testing in cardiovascular diseases. Current opinion in cardiology.
2010;
25
:
243-248
.
-
A.
Huerta,
B.
López,
S.
Ravassa,
G.
San José,
R.
Querejeta,
Ó.
Beloqui,
E.
Zubillaga,
G.
Rábago,
C.
Brugnolaro,
J.
Díez.
Association of cystatin C with heart failure with preserved ejection fraction in elderly hypertensive patients: potential role of altered collagen metabolism. Journal of hypertension.
2016;
34
:
130-138
.
-
K.R.
Hutchinson,
J.A.
Stewart,
P.A.
Lucchesi.
Extracellular matrix remodeling during the progression of volume overload-induced heart failure. Journal of molecular and cellular cardiology.
2010;
48
:
564-569
.
-
T.F.
Imran,
H.J.
Shin,
N.
Mathenge,
F.
Wang,
B.
Kim,
J.
Joseph,
J.M.
Gaziano,
L.
Djoussé.
Meta-Analysis of the Usefulness of Plasma Galectin-3 to Predict the Risk of Mortality in Patients With Heart Failure and in the General Population. The American Journal of Cardiology.
2017;
119
:
57-64
.
-
F.
Jansen,
G.
Nickenig,
N.
Werner.
Extracellular Vesicles in Cardiovascular Disease. Circulation research.
2017;
120
:
1649-1657
.
-
S.
Kang,
L.
Fan,
M.
Chen,
J.
Li,
Z.
Liu.
Relationship of high-sensitivity C-reactive protein concentrations and systolic heart failure. Current vascular pharmacology.
2017;
10.2174/1570161115666170404121619
.
-
T.
Kempf,
K.C.
Wollert.
Growth differentiation factor-15: a new biomarker in cardiovascular disease. Herz.
2009;
34
:
594-599
.
-
H.
Kim,
H.J.
Yoon,
H.S.
Park,
Y.K.
Cho,
C.W.
Nam,
S.H.
Hur,
Y.N.
Kim,
K.B.
Kim.
Potentials of cystatin C and uric acid for predicting prognosis of heart failure. Congestive Heart Failure.
2013;
19
:
123-129
.
-
T.-H.
Kim,
H.
Kim,
I.-C.
Kim.
The potential of cystatin-C to evaluate the prognosis of acute heart failure: A comparative study. Acute Cardiac Care.
2015;
17
:
72-76
.
-
T.
Kitai,
Y.-H.
Kim,
K.
Kiefer,
R.
Morales,
A.G.
Borowski,
J.L.
Grodin,
W.W.
Tang.
Circulating intestinal fatty acid-binding protein (I-FABP) levels in acute decompensated heart failure. Clinical Biochemistry.
2017;
50
:
491-495
.
-
I.C.
Kolder,
M.
Michels,
I.
Christiaans,
F.J.
Ten Cate,
D.
Majoor-Krakauer,
A.H.
Danser,
R.H.
Lekanne Deprez,
M.
Tanck,
A.A.
Wilde,
C.R.
Bezzina.
The role of renin-angiotensin-aldosterone system polymorphisms in phenotypic expression of MYBPC3-related hypertrophic cardiomyopathy. European journal of human genetics : EJHG.
2012;
20
:
1071-1077
.
-
V.
Krane,
B.
Genser,
M.E.
Kleber,
C.
Drechsler,
W.
März,
G.
Delgado,
B.
Allolio,
C.
Wanner,
W.
Fenske.
Copeptin Associates with Cause-Specific Mortality in Patients with Impaired Renal Function: Results from the LURIC and the 4D Study. Clinical Chemistry.
2017;
63
:
997-1007
.
-
M.
Krintus,
M.
Kozinski,
T.
Fabiszak,
J.
Kubica,
M.
Panteghini,
G.
Sypniewska.
Establishing reference intervals for galectin-3 concentrations in serum requires careful consideration of its biological determinants. Clinical Biochemistry.
2017;
50
:
599-604
.
-
M.
Ledwidge,
J.
Gallagher,
C.
Conlon,
E.
Tallon,
E.
O’Connell,
I.
Dawkins,
C.
Watson,
R.
O’Hanlon,
M.
Bermingham,
A.
Patle.
Natriuretic peptide-based screening and collaborative care for heart failure: the STOP-HF randomized trial. Jama.
2013;
310
:
66-74
.
-
J.
Li,
K.
Yousefi,
W.
Ding,
J.
Singh,
L.A.
Shehadeh.
Osteopontin RNA aptamer can prevent and reverse pressure overload-induced heart failure. Cardiovascular research.
2017;
113
:
633-643
.
-
J.
Löfsjögård,
T.
Kahan,
J.
Díez,
B.
López,
A.
González,
S.
Ravassa,
M.
Mejhert,
M.
Edner,
H.
Persson.
Usefulness of Collagen Carboxy-Terminal Propeptide and Telopeptide to Predict Disturbances of Long-Term Mortality in Patients≥ 60 Years With Heart Failure and Reduced Ejection Fraction. The American Journal of Cardiology.
2017;
119
:
2042-2048
.
-
J.
Löfsjögård,
H.
Persson,
J.
Díez,
B.
López,
A.
González,
M.
Edner,
M.
Mejhert,
T.
Kahan.
Atrial fibrillation and biomarkers of myocardial fibrosis in heart failure. Scandinavian Cardiovascular Journal.
2014;
48
:
299-303
.
-
L.R.
Lopes,
P.M.
Elliott.
Genetics of heart failure. Biochimica et biophysica acta.
2013;
1832
:
2451-2461
.
-
A.
Luchner,
S.
Von Haehling,
C.
Holubarsch,
T.
Keller,
F.
Knebel,
C.
Zugck,
U.
Laufs.
Indications and Clinical Implications of the Use of the Cardiac Markers BNP and NT-proBNP. Deutsche medizinische Wochenschrift (1946).
2017;
142
:
346
.
-
A.S.
Maisel,
S.
Di Somma.
Do we need another heart failure biomarker: focus on soluble suppression of tumorigenicity 2 (sST2). European Heart Journal.
2016;
ehw462
.
-
V.
Malek,
A.B.
Gaikwad.
Neprilysin inhibitors: A new hope to halt the diabetic cardiovascular and renal complications?. Biomedicine & pharmacotherapy = Biomedecine & pharmacotherapie.
2017;
90
:
752-759
.
-
S.
Masson,
R.
Latini,
I.S.
Anand.
An update on cardiac troponins as circulating biomarkers in heart failure. Current heart failure reports.
2010;
7
:
15-21
.
-
D.M.
McNamara,
A.L.
Taylor,
S.W.
Tam,
M.
Worcel,
C.W.
Yancy,
K.
Hanley-Yanez,
J.N.
Cohn,
A.M.
Feldman.
G-protein beta-3 subunit genotype predicts enhanced benefit of fixed-dose isosorbide dinitrate and hydralazine: results of A-HeFT. JACC: Heart Failure.
2014;
2
:
551-557
.
-
W.C.
Meijers,
A.R.
van der Velde,
A.C.
Muller Kobold,
J.
Dijck-Brouwer,
A.H.
Wu,
A.
Jaffe,
R.A.
de Boer.
Variability of biomarkers in patients with chronic heart failure and healthy controls. European Journal of Heart Failure.
2016;
19
:
357-365
.
-
Ò.
Miró,
B.
González de la Presa,
P.
Herrero-Puente,
R.
Fernández Bonifacio,
M.
Möckel,
C.
Mueller,
G.
Casals,
S.
Sandalinas,
P.
Llorens,
F.J.
Martín-Sánchez.
The GALA study: relationship between galectin-3 serum levels and short-and long-term outcomes of patients with acute heart failure. Biomarkers.
2017;
10.1080/1354750X
:
1352017-1319421
.
-
Y.
Moayedi,
H.J.
Ross.
Advances in heart failure: a review of biomarkers, emerging pharmacological therapies, durable mechanical support and telemonitoring. Clinical science.
2017;
(London
:
England : 1979) 131, 553-566
.
-
N.G.
Morgenthaler,
J.
Struck,
C.
Alonso,
A.
Bergmann.
Assay for the measurement of copeptin, a stable peptide derived from the precursor of vasopressin. Clinical chemistry.
2006;
52
:
112-119
.
-
V.
Nagarajan,
A.V.
Hernandez,
W.W.
Tang.
Prognostic value of cardiac troponin in chronic stable heart failure: a systematic review. Heart, heartjnl-2012-301779.
2012
.
-
M.
Nakanishi,
K.
Nakao,
L.
Kumasaka,
T.
Arakawa,
S.
Fukui,
T.
Ohara,
M.
Yanase,
T.
Noguchi,
S.
Yasuda,
Y.
Goto.
Improvement in Exercise Capacity by Exercise Training Associated With Favorable Clinical Outcomes in Advanced Heart Failure With High B-Type Natriuretic Peptide Level. Circulation Journal.
2017;
CJ-16-1268
.
-
K.E.
Nelveg-Kristensen,
M.
Busk Madsen,
C.
Torp-Pedersen,
L.
Kober,
M.
Egfjord,
H.
Berg Rasmussen,
P.
Riis Hansen.
Pharmacogenetic Risk Stratification in Angiotensin-Converting Enzyme Inhibitor-Treated Patients with Congestive Heart Failure: A Retrospective Cohort Study. PloS one.
2015;
10
:
e0144195
.
-
S.H.
Nymo,
P.
Aukrust,
J.
Kjekshus,
J.J.
McMurray,
J.G.
Cleland,
J.
Wikstrand,
P.
Muntendam,
U.
Wienhues-Thelen,
R.
Latini,
E.T.
Askevold.
Limited Added Value of Circulating Inflammatory Biomarkers in Chronic Heart Failure. JACC Heart failure.
2017;
5
:
256-264
.
-
H.
Okazaki,
A.
Shirakabe,
N.
Kobayashi,
N.
Hata,
T.
Shinada,
M.
Matsushita,
Y.
Yamamoto,
Y.
Shibata,
J.
Shibuya,
R.
Shiomura.
Are atherosclerotic risk factors associated with a poor prognosis in patients with hyperuricemic acute heart failure? The evaluation of the causal dependence of acute heart failure and hyperuricemia. Heart and Vessels.
2017;
32
:
436-445
.
-
H.
Okazaki,
A.
Shirakabe,
N.
Kobayashi,
N.
Hata,
T.
Shinada,
M.
Matsushita,
Y.
Yamamoto,
J.
Shibuya,
R.
Shiomura,
S.
Nishigoori.
The prognostic impact of uric acid in patients with severely decompensated acute heart failure. Journal of cardiology.
2016;
68
:
384-391
.
-
Y.
Otaki,
T.
Watanabe,
D.
Kinoshita,
M.
Yokoyama,
T.
Takahashi,
T.
Toshima,
T.
Sugai,
T.
Murase,
T.
Nakamura,
S.
Nishiyama.
Association of plasma xanthine oxidoreductase activity with severity and clinical outcome in patients with chronic heart failure. International Journal of Cardiology.
2017;
228
:
151-157
.
-
P.
Ponikowski,
A.A.
Voors,
S.D.
Anker,
H.
Bueno,
J.G.
Cleland,
A.J.
Coats,
V.
Falk,
J.R.
González-Juanatey,
V.-P.
Harjola,
E.A.
Jankowska.
2016 ESC Guidelines for the diagnosis and treatment of acute and chronic heart failure: The Task Force for the diagnosis and treatment of acute and chronic heart failure of the European Society of Cardiology (ESC) Developed with the special contribution of the Heart Failure Association (HFA) of the ESC. European heart journal.
2016;
37
:
2129-2200
.
-
A.-C.
Pouleur.
Which biomarkers do clinicians need for diagnosis and management of heart failure with reduced ejection fraction?. Clinica Chimica Acta.
2015;
443
:
9-16
.
-
P.H.
Puente,
B.P.
García,
M.G.
García,
J.
Jacob,
F.J.
Martín-Sánchez,
D.
Pascual-Figal,
H.
Bueno,
V.
Gil,
P.
Llorens,
J.
Vázquez-Alvarez.
Predictive capacity of a multimarker strategy to determine short-term mortality in patients attending a hospital emergency department for acute heart failure. BIO-EAHFE study. Clinica Chimica Acta.
2017;
466
:
22-30
.
-
H.-Y.
Qian,
J.
Huang,
Y.-J.
Yang,
Y.-M.
Yang,
Z.-Z.
Li,
J.-M.
Zhang.
Heart-type Fatty Acid Binding Protein in the Assessment of Acute Pulmonary Embolism. The American Journal of the Medical Sciences.
2016;
352
:
557-562
.
-
M.M.
Reiner,
W.E.
Khoury,
M.B.
Canales,
R.A.
Chmielewski,
K.
Patel,
M.C.
Razzante,
C.O.
Cloughtery,
D.Y.
Rowland.
Procalcitonin as a Biomarker for Predicting Amputation Level in Lower Extremity Infections. The Journal of Foot and Ankle Surgery.
2017;
56
:
484-491
.
-
H.
Remde,
A.
Dietz,
R.
Emeny,
A.
Riester,
A.
Peters,
T.
de Las Heras Gala,
C.
Then,
J.
Seissler,
F.
Beuschlein,
M.
Reincke.
The cardiovascular markers copeptin and high-sensitive C-reactive protein decrease following specific therapy for primary aldosteronism. Journal of hypertension.
2016;
34
:
2066-2073
.
-
M.
Rienks,
A.-P.
Papageorgiou.
Novel regulators of cardiac inflammation: matricellular proteins expand their repertoire. Journal of molecular and cellular cardiology.
2016;
91
:
172-178
.
-
J.A.
Ryu,
J.H.
Yang,
D.
Lee,
C.M.
Park,
G.Y.
Suh,
K.
Jeon,
J.
Cho,
S.Y.
Baek,
K.C.
Carriere,
C.R.
Chung.
Clinical Usefulness of Procalcitonin and C-Reactive Protein as Outcome Predictors in Critically Ill Patients with Severe Sepsis and Septic Shock. PloS one.
2015;
10
:
e0138150
.
-
I.
Sahin,
B.
Gungor,
B.
Ozkaynak,
F.
Uzun,
S.H.
Küçük,
I.I.
Avci,
E.
Ozal,
B.
Ayça,
S.
Cetın,
E.
Okuyan.
Higher copeptin levels are associated with worse outcome in patients with hypertrophic cardiomyopathy. Clinical Cardiology.
2016;
40
:
32-37
.
-
S.S.
Sakamuri,
A.
Takawale,
R.
Basu,
P.W.
Fedak,
D.
Freed,
C.
Sergi,
G.Y.
Oudit,
Z.
Kassiri.
Differential impact of mechanical unloading on structural and nonstructural components of the extracellular matrix in advanced human heart failure. Translational Research.
2016;
172
:
30-44
.
-
A.
Savic-Radojevic,
M.
Pljesa-Ercegovac,
M.
Matic,
D.
Simic,
S.
Radovanovic,
T.
Simic.
Chapter Four-Novel Biomarkers of Heart Failure. Advances in Clinical Chemistry.
2017;
79
:
93-152
.
-
L.
Simon,
F.
Gauvin,
D.K.
Amre,
P.
Saint-Louis,
J.
Lacroix.
Serum procalcitonin and C-reactive protein levels as markers of bacterial infection: a systematic review and meta-analysis. Clinical infectious diseases.
2004;
39
:
206-217
.
-
M.I.
Smaradottir,
V.
Ritsinger,
V.
Gyberg,
A.
Norhammar,
P.
Näsman,
L.G.
Mellbin.
Copeptin in patients with acute myocardial infarction and newly detected glucose abnormalities-A marker of increased stress susceptibility? A report from the Glucose in Acute Myocardial Infarction cohort. Diabetes and Vascular Disease Research.
2017;
14
:
69-76
.
-
V.
Srivatsan,
M.
George,
E.
Shanmugam.
Utility of galectin-3 as a prognostic biomarker in heart failure: where do we stand?. European journal of preventive cardiology.
2015;
22
:
1096-1110
.
-
M.E.
Sutter,
A.
Gaedigk,
T.E.
Albertson,
J.
Southard,
K.P.
Owen,
L.D.
Mills,
D.B.
Diercks.
Polymorphisms in CYP2D6 may predict methamphetamine related heart failure. Clinical toxicology.
2013;
(Philadelphia
:
Pa) 51, 540-544
.
-
P.R.
Taub,
K.C.
Borden,
A.
Fard,
A.
Maisel.
Role of biomarkers in the diagnosis and prognosis of acute kidney injury in patients with cardiorenal syndrome. Expert review of cardiovascular therapy.
2012;
10
:
657-667
.
-
P.
Teekakirikul,
M.A.
Kelly,
H.L.
Rehm,
N.K.
Lakdawala,
B.H.
Funke.
Inherited cardiomyopathies: molecular genetics and clinical genetic testing in the postgenomic era. The Journal of Molecular Diagnostics.
2013;
15
:
158-170
.
-
L.Y.L.
Teo,
R.T.
Moran,
W.W.
Tang.
Evolving approaches to genetic evaluation of specific cardiomyopathies. Current heart failure reports.
2015;
12
:
339-349
.
-
J.
Tromp,
M.A.
Khan,
I.T.
Klip,
S.
Meyer,
R.A.
de Boer,
T.
Jaarsma,
H.
Hillege,
D.J.
van Veldhuisen,
P.
van der Meer,
A.A.
Voors.
Biomarker Profiles in Heart Failure Patients With Preserved and Reduced Ejection Fraction. Journal of the American Heart Association.
2017;
6
:
e003989
.
-
D.N.
Tziakas,
G.K.
Chalikias,
D.
Stakos,
S.V.
Chatzikyriakou,
D.
Papazoglou,
K.
Mitrousi,
A.
Lantzouraki,
A.
Thomaidi,
H.
Boudoulas,
S.
Konstantinides.
Independent and additive prognostic ability of serum carboxy-terminal telopeptide of collagen type-I in heart failure patients: a multi-marker approach with high-negative predictive value to rule out long-term adverse events. European journal of preventive cardiology.
2012;
19
:
62-71
.
-
I.
Valiente-Alandi,
A.E.
Schafer,
B.C.
Blaxall.
Extracellular matrix-mediated cellular communication in the heart. Journal of molecular and cellular cardiology.
2016;
91
:
228-237
.
-
N.
Wettersten,
A.S.
Maisel.
Biomarkers for heart failure: An update for practitioners of internal medicine. The American journal of medicine.
2016;
129
:
560-567
.
-
C.
Wojciechowska,
E.
Romuk,
E.
Nowalany-Kozielska,
W.
Jacheć.
Serum Galectin-3 and ST2 as predictors of unfavorable outcome in stable dilated cardiomyopathy patients. Hellenic Journal of Cardiology.
2017;
S1109-9666
:
30249-30244
.
-
P.C.
Wong,
J.
Guo,
A.
Zhang.
The renal and cardiovascular effects of natriuretic peptides. Advances in physiology education.
2017;
41
:
179-185
.
-
C.K.
Wu,
J.L.
Luo,
C.T.
Tsai,
Y.T.
Huang,
C.L.
Cheng,
J.K.
Lee,
L.Y.
Lin,
J.W.
Lin,
J.J.
Hwang,
F.T.
Chiang.
Demonstrating the pharmacogenetic effects of angiotensin-converting enzyme inhibitors on long-term prognosis of diastolic heart failure. The pharmacogenomics journal.
2010;
10
:
46-53
.
-
C.K.
Wu,
J.L.
Luo,
X.M.
Wu,
C.T.
Tsai,
J.W.
Lin,
J.J.
Hwang,
J.L.
Lin,
C.D.
Tseng,
F.T.
Chiang.
A propensity score-based case-control study of renin-angiotensin system gene polymorphisms and diastolic heart failure. Atherosclerosis.
2009;
205
:
497-502
.
-
J.J.
Yan,
Y.
Lu,
Z.P.
Kuai,
Y.H.
Yong.
Predictive value of plasma copeptin level for the risk and mortality of heart failure: a meta-analysis.. Journal of Cellular and Molecular Medicine.
2017;
10.1111/jcmm.13102
.
-
C.W.
Yancy,
M.
Jessup,
B.
Bozkurt,
J.
Butler,
D.E. Jr.
Casey,
M.M.
Colvin,
M.H.
Drazner,
G.S.
Filippatos,
G.C.
Fonarow,
M.M.
Givertz.
2017 ACC/AHA/HFSA Focused Update of the 2013 ACCF/AHA Guideline for the Management of Heart Failure: A Report of the American College of Cardiology/American Heart Association Task Force on Clinical Practice Guidelines and the Heart Failure Society of America. Journal of cardiac failure.
2017;
10.1016/j.cardfail.2017.1004.1014
.
-
J.
Yang,
W.W.
Xu,
S.J.
Hu.
Heart failure: advanced development in genetics and epigenetics. BioMed research international.
2015;
2015
:
352734
.
-
V.L.
Yip,
M.
Pirmohamed.
Expanding role of pharmacogenomics in the management of cardiovascular disorders. American journal of cardiovascular drugs :.
2013;
drugs
:
devices, and other interventions 13, 151-162
.
-
B.
Yu,
Y.
Zheng,
D.
Alexander,
T.A.
Manolio,
A.
Alonso,
J.A.
Nettleton,
E.
Boerwinkle.
Genome-wide association study of a heart failure related metabolomic profile among African Americans in the Atherosclerosis Risk in Communities (ARIC) study. Genetic epidemiology.
2013;
37
:
840-845
.
-
L.
Zhu,
Y.
Zou,
Y.
Wang,
X.
Luo,
K.
Sun,
H.
Wang,
L.
Jia,
Y.
Liu,
J.
Zou,
Z.
Yuan.
Prognostic Significance of Plasma High-Sensitivity C-Reactive Protein in Patients With Hypertrophic Cardiomyopathy. Journal of the American Heart Association.
2017;
6
:
e004529
.
Comments
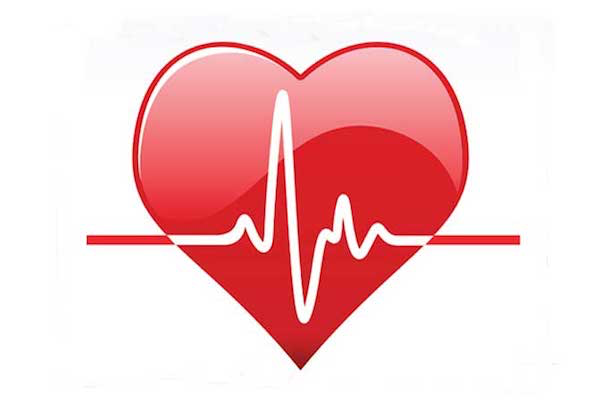
Downloads
Article Details
Volume & Issue : Vol 4 No 06 (2017)
Page No.: 1344-1373
Published on: 2017-06-24
Citations
Copyrights & License

This work is licensed under a Creative Commons Attribution 4.0 International License.
Search Panel
- HTML viewed - 8604 times
- Download PDF downloaded - 1715 times
- View Article downloaded - 21 times